A Priori Probability Definition Formula Example
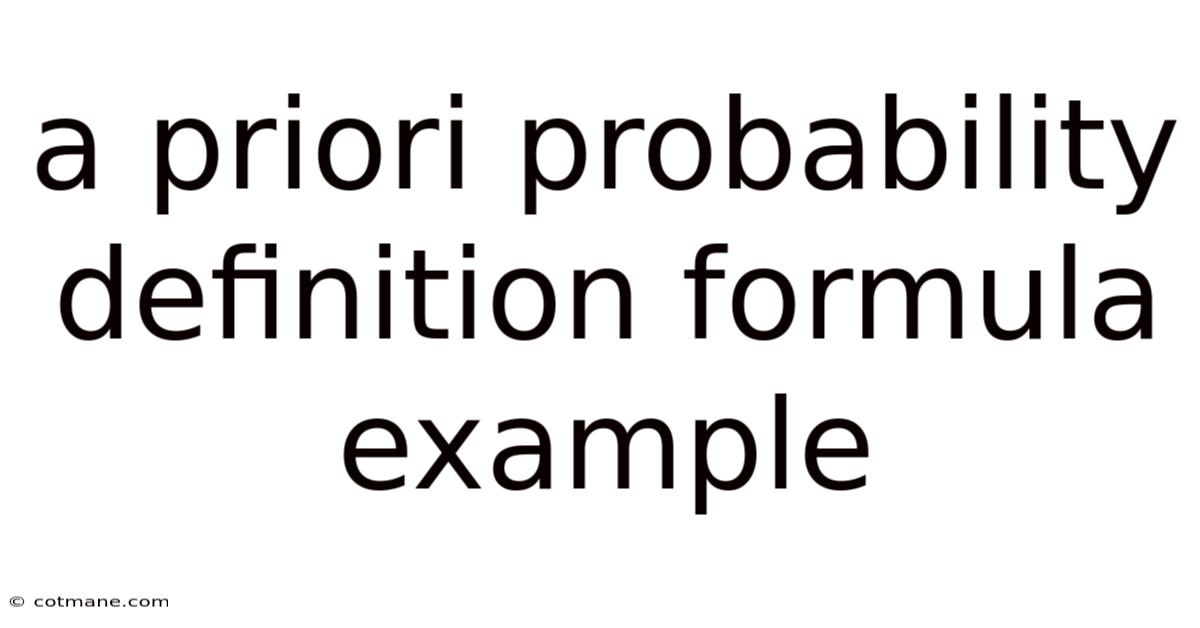
Discover more detailed and exciting information on our website. Click the link below to start your adventure: Visit Best Website meltwatermedia.ca. Don't miss out!
Table of Contents
Unveiling the Mysteries of A Priori Probability: Definition, Formula, and Examples
What makes a priori probability a cornerstone of modern probability theory?
A priori probability, a fundamental concept in probability and statistics, provides a powerful framework for understanding and predicting uncertain events based on prior knowledge and logical reasoning.
Editor’s Note: This comprehensive exploration of a priori probability has been published today.
Why A Priori Probability Matters
A priori probability, unlike its empirical counterpart (a posteriori probability), doesn't rely on observed data or experimental results. Instead, it leverages deductive reasoning and prior knowledge about the nature of the events to assign probabilities. This approach is particularly valuable in situations where:
- Empirical data is scarce or unavailable: In novel situations or when dealing with rare events, a priori probability offers a means of assigning probabilities based on logical deduction.
- Precise predictions are crucial: Applications in areas like risk assessment, decision-making under uncertainty, and game theory demand a robust and theoretically sound approach to probability calculation, which a priori probability provides.
- Theoretical models are being developed: A priori probability plays a vital role in the development and validation of theoretical models in diverse fields, including physics, engineering, and computer science. It forms the basis for establishing theoretical probabilities before empirical testing.
- Fairness and impartiality are paramount: In scenarios demanding unbiased assessments, such as game design or legal proceedings, a priori probability ensures that probabilities are derived from inherent characteristics rather than biased observations.
Overview of the Article
This article provides a comprehensive exploration of a priori probability, delving into its precise definition, the formula employed in its calculation, and diverse illustrative examples. Readers will gain a deep understanding of its theoretical underpinnings, its practical applications, and its crucial role in various disciplines. The article further explores the connections between a priori probability and other crucial concepts within probability theory, such as Bayes' Theorem and conditional probability.
Research and Effort Behind the Insights
The insights presented in this article are derived from a thorough review of seminal texts on probability theory, statistical analysis, and related fields. The explanations and examples are carefully constructed to ensure clarity and accessibility, while rigorous mathematical accuracy is maintained throughout. The information presented is supported by established principles and methodologies within the field of probability.
Key Aspects of A Priori Probability
Key Aspect | Description |
---|---|
Definition | Probability assigned based on prior knowledge and logical reasoning, independent of empirical observations. |
Formula | Depends on the specific problem; generally involves counting favorable outcomes and dividing by the total possible outcomes (classical definition). |
Classical Approach | Assumes all outcomes are equally likely. |
Subjective Approach | Allows for subjective judgments when equal likelihood is not assumed. |
Applications | Risk assessment, decision-making, game theory, theoretical model development, Bayesian inference. |
Let’s dive deeper into the key aspects of a priori probability, starting with its foundational principles and real-world applications.
Defining A Priori Probability
A priori probability, also known as classical probability, is the probability of an event based on prior knowledge of the conditions under which the event can occur. It's calculated by analyzing the possibilities before any experiment or observation. This approach fundamentally rests on the assumption that all possible outcomes are equally likely. This assumption is crucial and forms the basis of the classical definition.
The Formula: The classical approach utilizes a straightforward formula:
P(A) = (Number of favorable outcomes for event A) / (Total number of possible outcomes)
Where:
- P(A) represents the probability of event A.
- The numerator counts the instances where event A occurs.
- The denominator encompasses all possible outcomes of the event under consideration.
Examples of A Priori Probability
Let's illustrate the application of a priori probability through several examples:
Example 1: Rolling a Fair Die
Consider rolling a six-sided fair die. What is the probability of rolling a 3?
Since the die is fair, each outcome (1, 2, 3, 4, 5, 6) is equally likely.
- Number of favorable outcomes (rolling a 3): 1
- Total number of possible outcomes: 6
Therefore, P(rolling a 3) = 1/6
Example 2: Drawing a Card from a Standard Deck
What is the probability of drawing a King from a standard deck of 52 playing cards?
- Number of favorable outcomes (drawing a King): 4 (four Kings in the deck)
- Total number of possible outcomes: 52
Therefore, P(drawing a King) = 4/52 = 1/13
Example 3: Coin Toss
What is the probability of getting heads when flipping a fair coin?
- Number of favorable outcomes (getting heads): 1
- Total number of possible outcomes: 2 (heads or tails)
Therefore, P(getting heads) = 1/2
Example 4: Selecting a Marble from a Bag
A bag contains 5 red marbles and 3 blue marbles. What is the probability of selecting a red marble?
- Number of favorable outcomes (selecting a red marble): 5
- Total number of possible outcomes: 8 (5 red + 3 blue)
Therefore, P(selecting a red marble) = 5/8
Beyond the Classical Definition: Subjective A Priori Probability
While the classical definition forms the foundation of a priori probability, the assumption of equally likely outcomes doesn't always hold true in real-world scenarios. This limitation led to the development of a subjective approach to a priori probability. In this approach, probabilities are assigned based on expert judgment, prior knowledge, or a combination of both, even when outcomes aren't equally likely. This approach is often used in situations where a lack of complete information prevents the application of the classical method. For example, estimating the probability of a new product succeeding in the market often involves subjective judgment based on market analysis, competitor analysis, and expert opinion rather than a simple calculation of equally likely outcomes.
Exploring the Connection Between Bayesian Inference and A Priori Probability
Bayesian inference is a powerful statistical framework that leverages a priori probability in a fundamental way. Bayesian inference updates probabilities based on new evidence. The a priori probability, often referred to as the prior probability, represents the initial belief about the probability of an event before considering any new data. As new evidence becomes available, the prior probability is updated to produce a posterior probability, reflecting the revised belief after incorporating the new information. Bayes' Theorem provides the mathematical framework for this update.
Further Analysis of Bayesian Inference
Bayes' Theorem is expressed mathematically as:
P(A|B) = [P(B|A) * P(A)] / P(B)
Where:
- P(A|B) is the posterior probability of event A given that event B has occurred.
- P(B|A) is the likelihood of event B given that event A has occurred.
- P(A) is the prior probability of event A.
- P(B) is the prior probability of event B.
This formula showcases the crucial role of the a priori probability (P(A)) in shaping the posterior probability. The prior acts as the starting point, which is subsequently refined by incorporating the new evidence (P(B|A) and P(B)).
Illustrative Example of Bayesian Inference
Imagine a medical test for a rare disease. The prior probability of having the disease (P(A)) is very low, let's say 0.01%. The test is highly accurate, with a 99% chance of correctly identifying the disease (P(B|A)). However, it also has a 5% chance of producing a false positive (P(B|¬A)). If someone tests positive (B), what's the probability they actually have the disease (P(A|B))? Using Bayes' Theorem, we can calculate the posterior probability, taking into account both the prior probability and the test's performance. This example illustrates how Bayesian inference uses a priori probabilities to make informed decisions even when dealing with uncertain events.
FAQ Section
Q1: What is the difference between a priori and a posteriori probability?
A1: A priori probability is determined before any experiment or observation, based on prior knowledge and logical reasoning. A posteriori probability is calculated after an experiment or observation, based on the observed data.
Q2: Can a priori probability be applied to events with unequal likelihoods?
A2: While the classical definition assumes equal likelihood, the subjective approach allows for the assignment of a priori probabilities even when outcomes aren't equally likely.
Q3: How is a priori probability used in decision-making?
A3: A priori probabilities provide a basis for evaluating the potential outcomes of different decisions, allowing for more informed choices under uncertainty.
Q4: What are the limitations of a priori probability?
A4: The classical definition relies on the assumption of equally likely outcomes, which may not always be realistic. Furthermore, subjective a priori probabilities can be influenced by biases.
Q5: How does a priori probability relate to conditional probability?
A5: A priori probability provides the initial probability (prior) that is then updated using conditional probabilities in Bayesian inference.
Q6: Is a priori probability always accurate?
A6: The accuracy of a priori probability depends on the accuracy of the prior knowledge and assumptions used. It serves as a starting point that can be refined through further observation and data collection.
Practical Tips for Using A Priori Probability
- Clearly define the event: Ensure a precise definition of the event for which you are calculating the probability.
- Identify all possible outcomes: List all potential outcomes comprehensively.
- Determine the likelihood of each outcome: Assess whether outcomes are equally likely or if a subjective approach is necessary.
- Apply the formula: Use the classical formula (or a subjective approach) to calculate the probability.
- Consider context: Always consider the context and limitations of the a priori probability calculation.
- Refine with data: Use observed data to refine initial a priori probabilities, especially when dealing with subjective estimates.
- Consider expert opinions: When dealing with complex events, consult experts to inform the subjective assignment of probabilities.
- Communicate clearly: Clearly communicate the assumptions and methods used to obtain the a priori probability estimate.
Final Conclusion
A priori probability, while seemingly simple in its definition and formula, plays a surprisingly profound role in various fields. It provides a foundational approach to quantifying uncertainty, forming the basis for more sophisticated statistical methods like Bayesian inference. Its applications range from simple games of chance to complex decision-making processes in diverse fields like medicine, engineering, and finance. By understanding its strengths and limitations, we can harness the power of a priori probability to navigate uncertainty effectively and make informed choices. Further exploration of its intricacies will only deepen one's understanding of the fundamental principles governing probability and statistics.
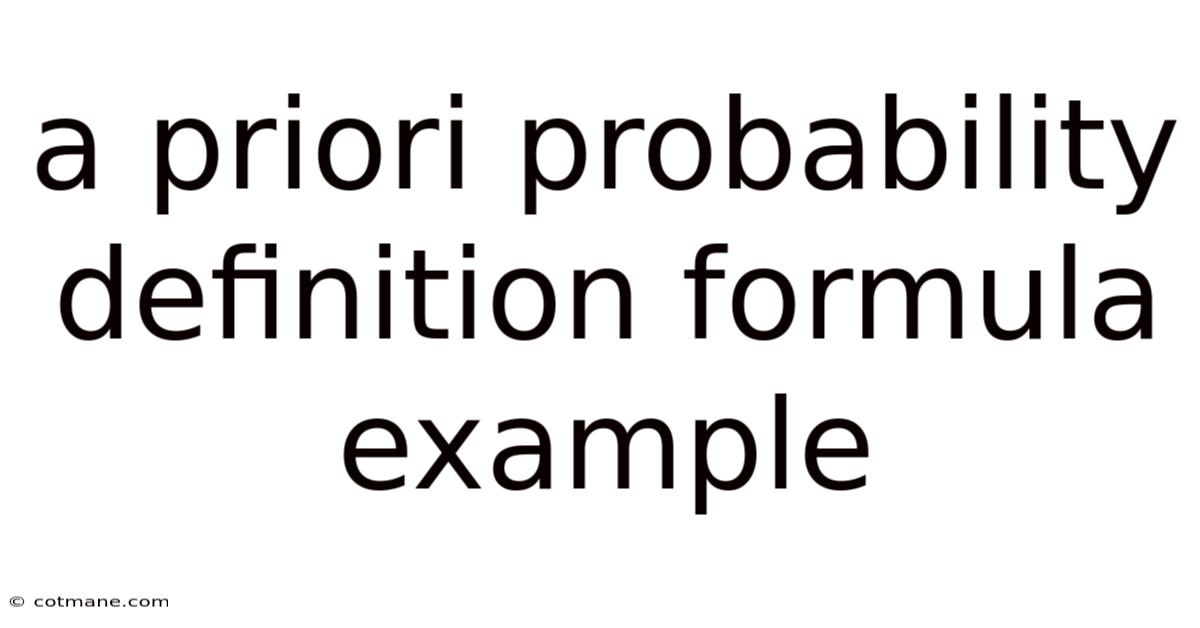
Thank you for visiting our website wich cover about A Priori Probability Definition Formula Example. We hope the information provided has been useful to you. Feel free to contact us if you have any questions or need further assistance. See you next time and dont miss to bookmark.
Also read the following articles
Article Title | Date |
---|---|
Make Money From Home | Apr 04, 2025 |
Adjustable Life Insurance Definition Pros Cons Vs Universal | Apr 04, 2025 |
Adventure Capitalist Defined | Apr 04, 2025 |
Annualized Rate Of Return Definition | Apr 04, 2025 |
What Are Otc Derivatives | Apr 04, 2025 |