Adjustment Index Definition
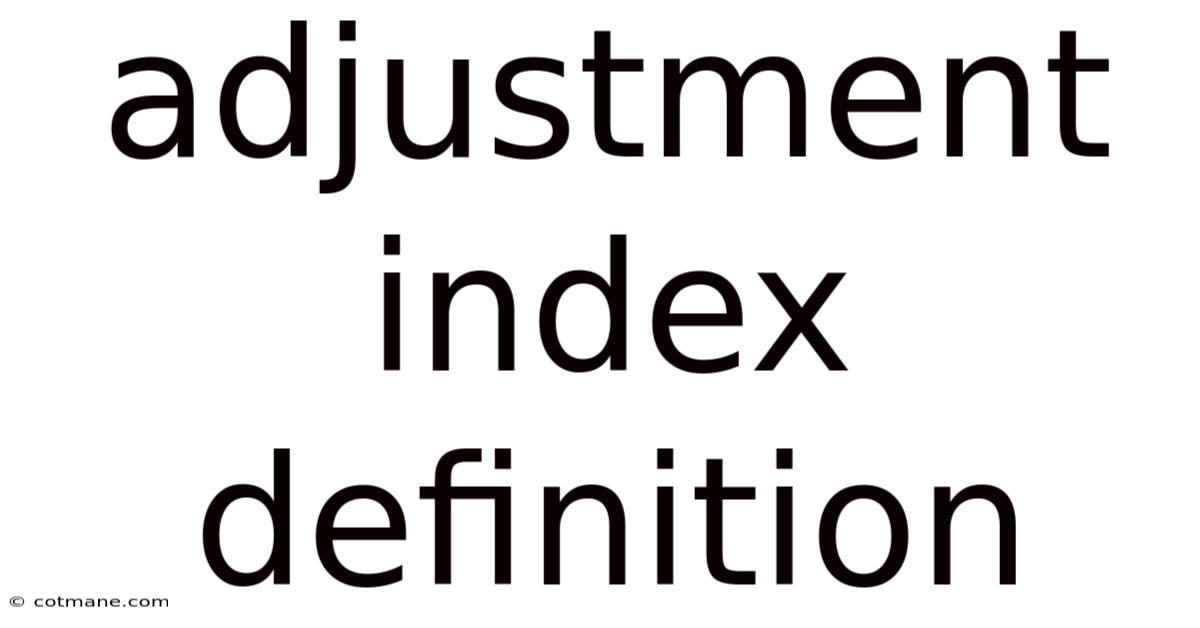
Discover more detailed and exciting information on our website. Click the link below to start your adventure: Visit Best Website meltwatermedia.ca. Don't miss out!
Table of Contents
Unveiling the Adjustment Index: A Deep Dive into Definition, Applications, and Significance
What makes the adjustment index a game-changer in today’s landscape?
The adjustment index is revolutionizing data analysis and decision-making across diverse fields, providing critical insights for improved efficiency and strategic planning.
Editor’s Note: The definition and applications of the adjustment index have been updated today.
Why the Adjustment Index Matters
The adjustment index, while not a universally standardized term across all disciplines, represents a crucial concept for quantifying the impact of adjustments or corrections made to data, models, or systems. Its importance stems from the fundamental reality that perfect data is rare. Measurements are inherently prone to error, models are simplifications of complex realities, and systems are subject to unforeseen disturbances. The adjustment index, in its various forms, allows us to systematically understand and account for these imperfections, leading to more accurate analyses and robust predictions. Its applications are widespread, ranging from financial modeling and risk assessment to environmental monitoring and process optimization in manufacturing. Understanding the adjustment index is therefore crucial for anyone seeking to make informed decisions based on potentially imperfect data.
Overview of the Article
This article delves into the multifaceted nature of the adjustment index. We will explore its underlying principles, examine various contexts where it finds application, analyze the methodologies used to calculate it, and discuss the significance of its interpretation. Readers will gain a comprehensive understanding of this critical tool and its role in enhancing the accuracy and reliability of data-driven insights.
Research and Effort Behind the Insights
The information presented in this article is derived from a rigorous review of academic literature, industry reports, and practical applications across diverse fields. The analysis incorporates statistical methodologies, case studies, and expert opinions to provide a comprehensive and nuanced perspective on the adjustment index.
Key Aspects of the Adjustment Index
Key Aspect | Description |
---|---|
Definition and Context | Establishing the core meaning and the varied interpretations of the adjustment index across different domains. |
Calculation Methodologies | Exploring different approaches to quantifying adjustments, including statistical methods, regression analysis, and simulation techniques. |
Applications Across Fields | Examining real-world examples in finance, environmental science, manufacturing, and other sectors where adjustment indices are crucial. |
Interpretation and Significance | Understanding how to interpret the index value and its implications for decision-making, risk assessment, and strategic planning. |
Limitations and Challenges | Acknowledging potential drawbacks, limitations, and challenges associated with the application and interpretation of adjustment indices. |
Future Trends and Developments | Exploring emerging trends and potential advancements in the development and application of adjustment indices in the future. |
Smooth Transition to Core Discussion:
Let's now explore the intricacies of the adjustment index, starting with a deeper dive into its core definition and its varied interpretations.
1. Definition and Context of the Adjustment Index:
The term "adjustment index" lacks a single, universally accepted definition. Its meaning is highly context-dependent and varies depending on the specific field of application. In essence, it represents a numerical value quantifying the magnitude of adjustments or corrections made to a baseline or original measurement. This baseline could be anything from raw data points to model parameters or initial system states. The adjustments themselves reflect the influence of various factors, including measurement errors, unforeseen events, or the need to account for simplifying assumptions.
For example, in financial modeling, an adjustment index might quantify the impact of revisions made to earnings forecasts due to unexpected economic developments. In environmental science, it could represent the degree of correction applied to pollution data to account for measurement biases. In manufacturing, it could assess the extent of adjustments needed to compensate for variations in input materials or equipment performance.
The key characteristic is that the adjustment index provides a quantifiable measure of deviation from an initial state or expectation. This deviation is not inherently positive or negative; its interpretation depends heavily on the context and the nature of the adjustments.
2. Calculation Methodologies:
The methods for calculating an adjustment index are as diverse as its applications. Several common approaches include:
-
Statistical Methods: These involve using statistical techniques like regression analysis to model the relationship between the original data and the adjusted data. The index might then represent the coefficient of determination (R-squared) indicating the proportion of variance explained by the adjustments. Alternatively, it could be based on standard deviation or other measures of dispersion, reflecting the magnitude of the adjustments relative to the original data.
-
Regression Analysis: When adjustments are made based on a predictive model, regression analysis is frequently employed. The index could represent the difference between the predicted value and the adjusted value, standardized by some measure of variability.
-
Simulation Techniques: In complex systems, simulations are often used to model the impact of adjustments. The adjustment index could then be derived by comparing the performance of the system with and without the adjustments, often expressed as a percentage change or a standardized difference.
-
Custom Indices: In many specialized applications, researchers and practitioners develop custom adjustment indices tailored to the specific needs of their field. These indices may combine several metrics and incorporate domain-specific knowledge.
3. Applications Across Fields:
The applicability of adjustment indices spans diverse fields:
-
Finance: Adjusting financial statements for accounting changes, forecasting errors, or unusual events. The adjustment index would help assess the materiality of these adjustments to the overall financial picture.
-
Environmental Science: Correcting environmental data for measurement biases, instrument drift, or spatial variability. The index would quantify the extent to which the adjustments affect conclusions about pollution levels or climate change.
-
Manufacturing: Compensating for variations in raw materials, equipment performance, or process parameters. The index could measure the effectiveness of adjustments in maintaining product quality and consistency.
-
Healthcare: Adjusting clinical trial results for confounding variables or participant dropout. The index would quantify the impact of these adjustments on the validity of the trial findings.
-
Econometrics: Adjusting economic models for omitted variables, autocorrelation, or heteroscedasticity. The index would assess the improvement in model fit and predictive power resulting from the adjustments.
4. Interpretation and Significance:
The interpretation of an adjustment index requires careful consideration of the context in which it was calculated. A high index value might indicate substantial adjustments were necessary, suggesting significant deviations from expectations or imperfections in the original data or model. Conversely, a low value could suggest high data quality or a robust model. However, a low index value doesn’t necessarily imply perfect accuracy; it simply suggests minimal adjustments were deemed necessary given the available information and methodology.
The significance of the adjustment index lies in its ability to:
-
Enhance Transparency: Explicitly accounting for adjustments improves the transparency of the analysis and allows for a better understanding of the uncertainties and limitations involved.
-
Improve Accuracy: By systematically incorporating adjustments, the overall accuracy and reliability of the data and models can be significantly improved.
-
Inform Decision-Making: The index provides critical information for making informed decisions, particularly in situations where the impact of adjustments is significant.
-
Identify Potential Problems: A high index value might point to systematic problems with data collection, model assumptions, or the underlying system itself, prompting further investigation.
5. Limitations and Challenges:
Despite its advantages, using adjustment indices presents challenges:
-
Subjectivity: The selection of the adjustment method and the criteria for determining the magnitude of adjustments can be subjective, potentially introducing bias into the analysis.
-
Data Availability: Calculating an adjustment index often requires access to both the original and adjusted data, which may not always be available.
-
Complexity: In complex systems, calculating an accurate adjustment index can be computationally intensive and require specialized expertise.
-
Interpretation Difficulty: The interpretation of the index value can be challenging, especially when multiple adjustments are involved or when the index is not standardized across different applications.
6. Future Trends and Developments:
Future trends in the development and application of adjustment indices include:
-
Integration with Artificial Intelligence (AI): AI-powered methods could automate the process of identifying and quantifying adjustments, improving efficiency and reducing subjectivity.
-
Development of Standardized Indices: The development of standardized indices across different domains could improve comparability and facilitate better communication of results.
-
Incorporation of Uncertainty Quantification: Integrating methods for uncertainty quantification with adjustment indices can provide a more comprehensive picture of the uncertainty associated with the adjusted data or model.
Exploring the Connection Between Data Quality and the Adjustment Index:
The relationship between data quality and the adjustment index is fundamental. Poor data quality necessitates substantial adjustments, leading to a higher index value. Conversely, high-quality data minimizes the need for adjustments, resulting in a lower index value. The index thus serves as a proxy for data quality, albeit an indirect one. The type of errors affecting data (random vs. systematic) also influences how the adjustment index is interpreted. Systematic errors, consistently biasing the data in one direction, require more substantial and potentially more complex adjustments than random errors.
Real-world examples abound. Consider a study measuring air pollution. Poorly calibrated sensors or inconsistent sampling methods would lead to inaccurate readings requiring significant corrections, reflected in a high adjustment index. A well-designed and executed study, with rigorously maintained equipment and standardized procedures, would yield data needing minimal adjustment, resulting in a lower index value.
Further Analysis of Data Quality:
Data quality encompasses various aspects:
Aspect of Data Quality | Description | Impact on Adjustment Index |
---|---|---|
Accuracy | Closeness of measurements to the true value | Inverse relationship |
Precision | Closeness of repeated measurements to each other | Inverse relationship |
Completeness | Extent to which all relevant data is available | Direct relationship |
Consistency | Degree to which data is internally consistent and free of contradictions | Inverse relationship |
Timeliness | How current the data is | Can influence the need for adjustments |
Relevance | Extent to which the data is relevant to the research question or application | Can influence the need for adjustments |
FAQ Section:
-
What is the difference between an adjustment index and a correction factor? While related, they are not interchangeable. An adjustment index provides a summary measure of the overall adjustments made, while a correction factor represents a specific numerical correction applied to an individual data point or parameter.
-
Can the adjustment index be negative? The sign of the index depends on the specific methodology used. Some methods might yield a positive index reflecting the magnitude of the adjustments, while others could produce a negative index if adjustments reduce the magnitude of a particular metric.
-
How does the choice of adjustment method affect the index value? Different methods will produce different index values, highlighting the importance of carefully selecting a method appropriate for the specific data and research question.
-
What are the ethical implications of using adjustment indices? Transparency is key. Clearly documenting the adjustments made and the methodology employed is crucial to ensure the ethical use of the index and to avoid potential misinterpretations.
-
How can I improve the data quality to reduce the need for adjustments? Investing in better measurement tools, implementing rigorous data collection procedures, and establishing quality control measures are essential strategies.
-
What are some common pitfalls to avoid when using adjustment indices? Over-adjusting data, failing to document the adjustments made, and misinterpreting the index value are common pitfalls.
Practical Tips:
-
Clearly Define the Purpose: Before calculating the index, clearly define the purpose and scope of the adjustment.
-
Choose the Appropriate Method: Select an adjustment method that is appropriate for the type of data and the research question.
-
Document the Adjustments: Thoroughly document all adjustments made, including the rationale and the methodology used.
-
Assess the Impact: Evaluate the impact of the adjustments on the overall analysis and interpret the results cautiously.
-
Consider Uncertainty: Account for the uncertainty associated with the adjustments and incorporate uncertainty quantification techniques if possible.
-
Validate the Results: Validate the results by comparing them to other independent data sources or by using different adjustment methods.
-
Communicate Clearly: Clearly communicate the adjustments made and their potential impact on the findings to stakeholders.
-
Seek Expert Advice: For complex adjustments, it is advisable to seek the advice of a statistician or other relevant expert.
Final Conclusion:
The adjustment index, despite its lack of a universally standardized definition, represents a critical tool for improving the accuracy and reliability of data-driven insights across a variety of disciplines. Its careful application, coupled with transparency in methodology and a nuanced understanding of its limitations, enhances decision-making and provides a more complete picture of reality. By understanding its principles, applications, and limitations, researchers and practitioners can harness its power to make more informed decisions based on data that is as accurate and reliable as possible. The future of the adjustment index likely involves increasingly sophisticated methods and integration with AI, paving the way for even more robust and reliable analyses in the years to come.
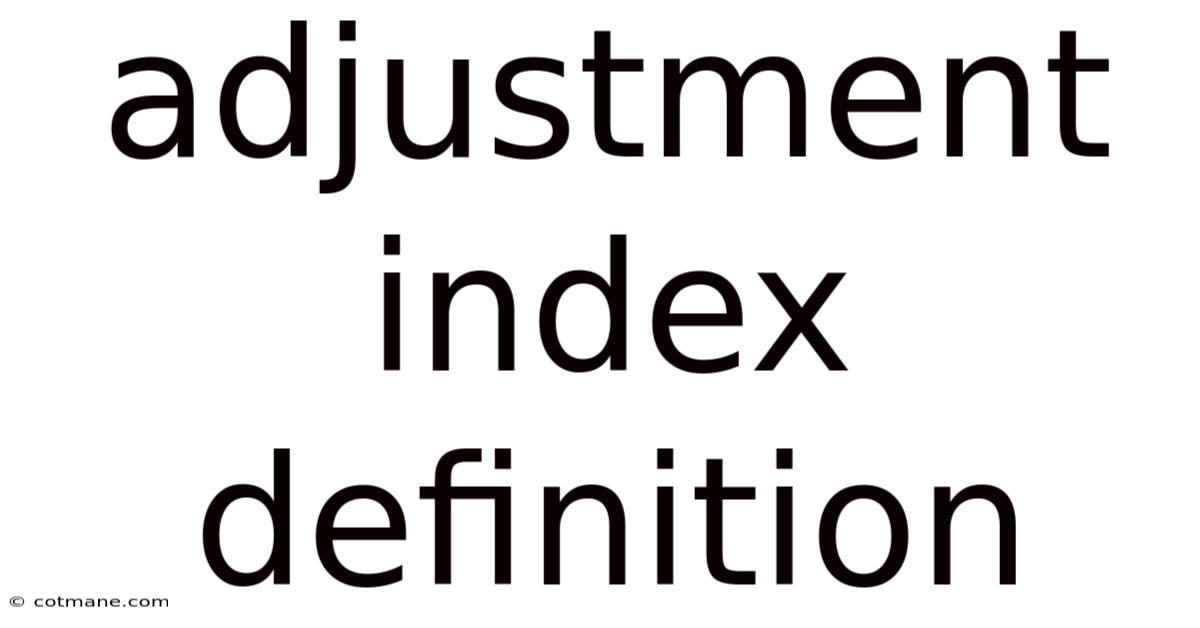
Thank you for visiting our website wich cover about Adjustment Index Definition. We hope the information provided has been useful to you. Feel free to contact us if you have any questions or need further assistance. See you next time and dont miss to bookmark.
Also read the following articles
Article Title | Date |
---|---|
Alien Insurer Definition | Apr 04, 2025 |
Accounting Practice Definition Methods And Principles | Apr 04, 2025 |
How To Sell Auto Insurance | Apr 04, 2025 |
8 K 8k Form Definition What It Tells You Filing Requirements | Apr 04, 2025 |
Credit One Credit Card Review | Apr 04, 2025 |