Runs Test Definition Types Uses And Benefits
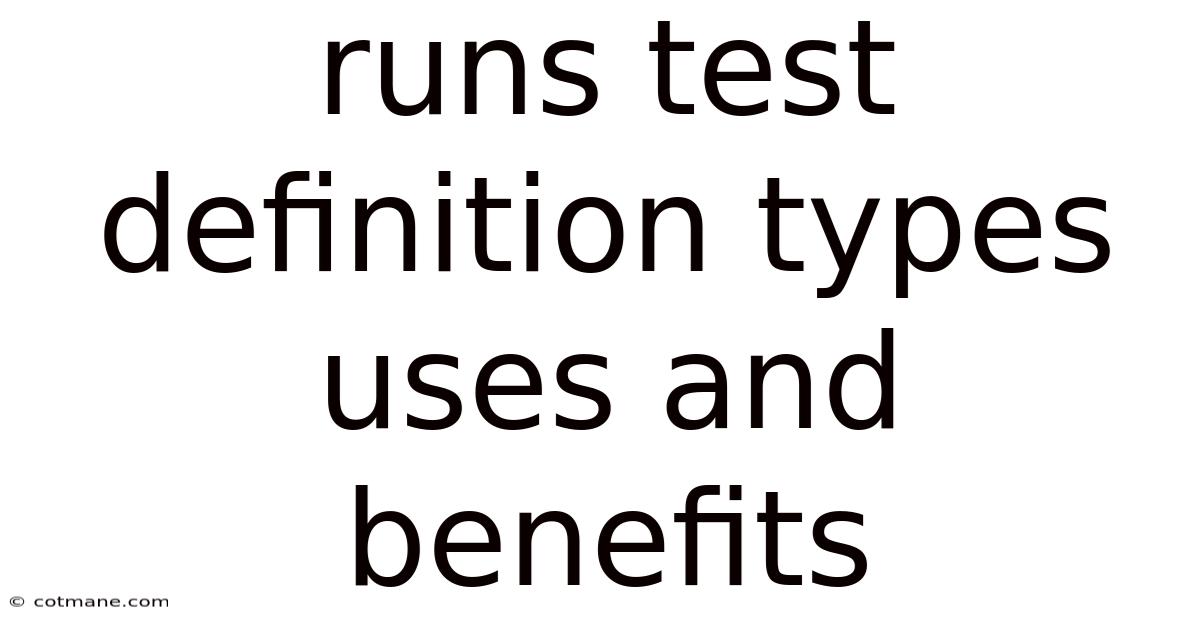
Discover more detailed and exciting information on our website. Click the link below to start your adventure: Visit Best Website meltwatermedia.ca. Don't miss out!
Table of Contents
Unveiling the Power of Runs Tests: Definition, Types, Uses, and Benefits
What makes the runs test a powerful statistical tool in today's data analysis landscape?
Runs tests are invaluable for identifying patterns and randomness in data sequences, providing crucial insights across diverse fields.
Editor's Note: This comprehensive guide to runs tests has been published today, offering up-to-date information and practical applications for data analysts and researchers.
Why Runs Tests Matter
Runs tests are non-parametric statistical tests used to determine whether the order of observations in a sequence is random. Unlike many other statistical tests that focus on the distribution of data, runs tests analyze the sequence of data points, looking for patterns or clusters that deviate from pure randomness. Their importance stems from their ability to detect subtle deviations from randomness that might be missed by other methods. This is particularly relevant in various fields, including:
- Quality Control: Detecting anomalies in manufacturing processes or product quality. Consistent runs of defects indicate a systemic problem, while alternating runs might suggest random variations.
- Financial Markets: Identifying trends and patterns in stock prices or other financial data. A series of consistently increasing or decreasing values might suggest a trend, whereas random fluctuations indicate a more stable market.
- Environmental Science: Analyzing time series data, such as rainfall or temperature fluctuations, to identify recurring patterns or shifts in climate.
- Medicine: Examining the sequence of successes and failures in clinical trials to assess the effectiveness of a treatment.
- Genetics: Analyzing DNA sequences to identify regions of unusual clustering or randomness, which may be indicative of mutations or other genetic variations.
Overview of the Article
This article will delve into the intricacies of runs tests, exploring their definition, various types, practical applications, and significant benefits. We will examine the underlying principles, different approaches for conducting the test, and interpret the results to extract valuable insights. Readers will gain a comprehensive understanding of how to apply runs tests effectively to analyze sequential data and make data-driven decisions.
Research and Effort Behind the Insights
This article draws upon extensive research from statistical literature, encompassing seminal works on non-parametric statistics and numerous case studies illustrating the practical applications of runs tests across diverse domains. The information presented reflects a rigorous review of established methodologies and their interpretations.
Key Aspects of Runs Tests
Key Aspect | Description |
---|---|
Definition | A statistical test for randomness in a sequence of data. It examines the number of runs (sequences of identical values) in the data. |
Types of Runs Tests | Several types exist, including the Wald-Wolfowitz runs test for two samples and tests for runs above and below the median. |
Assumptions | Primarily, the data should be ordinal (at least rankable). Specific assumptions vary based on the type of runs test being employed. |
Test Statistic | The number of runs (R) in the sequence is the primary test statistic. Different test statistics exist based on the specific runs test. |
Interpretation | A significantly low or high number of runs suggests non-randomness, while a number within the expected range indicates randomness. |
Applications | Diverse applications across quality control, finance, environmental science, medicine, and genetics, to name a few. |
Let's dive deeper into the key aspects of runs tests, starting with their foundational principles and real-world applications.
The Basics of Runs Tests: Understanding Runs
At the heart of any runs test lies the concept of a "run." A run is defined as a consecutive sequence of identical observations. For example, consider the sequence: A, A, B, B, B, A, A, A, B. This sequence contains four runs: two runs of A's, one run of B's, and another run of A's. The runs test assesses the number of runs (R) observed in a sequence and compares it to the expected number of runs under the null hypothesis of randomness. If the observed number of runs deviates significantly from the expected number, it suggests that the sequence is non-random.
Types of Runs Tests
Several types of runs tests exist, each tailored to specific data characteristics and research questions:
-
Wald-Wolfowitz Runs Test: This is the most common type, used to test the randomness of a sequence of two categories (e.g., successes and failures, above and below the median). The test statistic is the number of runs (R), and its distribution is approximated using a normal distribution for larger sample sizes.
-
Runs Test Above and Below the Median: This test assesses the randomness of a sequence by comparing each observation to the median. Runs of observations above the median and runs of observations below the median are counted.
-
Runs Test for Trend: Variations exist to specifically detect trends in data. These tests are often more sensitive to monotonic trends (consistent increase or decrease) than the standard Wald-Wolfowitz test.
-
Modifications for Tied Observations: In datasets with tied observations (identical values), modifications to the standard runs test are often necessary to account for the impact of ties on the number of runs.
Conducting a Runs Test: A Step-by-Step Guide (Wald-Wolfowitz)
-
Define the Categories: Identify the two categories that will form the basis of the runs test (e.g., success/failure, above/below median).
-
Count the Runs: Determine the number of runs (R) in the sequence of observations.
-
Calculate the Expected Number of Runs: This involves using formulas that depend on the number of observations in each category (n1 and n2). The formula for the expected number of runs (μR) is: μR = (2n1n2 / (n1 + n2)) + 1
-
Calculate the Standard Deviation of Runs: The standard deviation (σR) is calculated using the formula: σR = √((2n1n2(2n1n2 - n1 - n2)) / ((n1 + n2)²(n1 + n2 - 1)))
-
Calculate the Test Statistic: The test statistic (Z) is calculated as: Z = (R - μR) / σR
-
Determine the p-value: Using a standard normal distribution table or statistical software, determine the p-value associated with the calculated Z-statistic.
-
Interpret the Results: If the p-value is less than the chosen significance level (e.g., 0.05), the null hypothesis of randomness is rejected, suggesting that the sequence is non-random.
Interpreting Results and Addressing Non-Randomness
A significant p-value indicates that the observed number of runs is significantly different from what would be expected under the assumption of randomness. A low number of runs suggests clustering or a trend, while a high number of runs might indicate an alternating pattern. The nature of the non-randomness needs to be further investigated using other statistical methods or domain expertise.
Exploring the Connection Between Autocorrelation and Runs Tests
Autocorrelation measures the correlation between a data point and its lagged values (previous data points). High autocorrelation suggests patterns or trends, often manifesting as long runs in a runs test. Conversely, low autocorrelation implies less predictable data, often associated with a greater number of runs close to the expected value. Both runs tests and autocorrelation analysis can provide complementary insights into the structure of time-series data.
Further Analysis of Autocorrelation and its Implications
Autocorrelation, particularly in time series data, can significantly influence the results of runs tests. Positive autocorrelation leads to fewer runs than expected, suggesting a trend. Negative autocorrelation produces more runs than expected, often indicating an alternating pattern. Understanding and addressing autocorrelation is crucial for accurate interpretation of runs test results. Techniques like differencing (subtracting consecutive data points) can sometimes reduce autocorrelation before applying a runs test.
Benefits of Using Runs Tests
-
Simplicity and Ease of Use: Runs tests are relatively simple to understand and implement, requiring minimal statistical expertise.
-
Non-parametric Nature: They don't assume any specific distribution of the data, making them applicable to a wide range of data types.
-
Sensitivity to Patterns: Runs tests are highly sensitive to detecting both clustering and alternating patterns in sequential data.
-
Visual Interpretation: The concept of runs is intuitively grasped, allowing for easy visual interpretation of results.
-
Complementary to Other Tests: Runs tests can be used in conjunction with other statistical methods to provide a more comprehensive understanding of data structure.
FAQ Section
Q1: What are the limitations of runs tests?
A1: Runs tests are primarily sensitive to detecting patterns in the order of observations. They may not be as powerful as other tests for detecting other forms of non-randomness, such as variations in the distribution of the data.
Q2: Can runs tests be used with continuous data?
A2: Yes, but continuous data needs to be categorized (e.g., above/below the median) before applying a runs test.
Q3: What if my data has many ties?
A3: Modifications to the standard runs test are needed to accommodate tied observations. Specialized runs tests or alternative methods might be more appropriate.
Q4: How do I choose the appropriate type of runs test?
A4: The choice depends on your research question and data characteristics. The Wald-Wolfowitz test is commonly used for two categories, while the runs test above and below the median is suitable for analyzing continuous data.
Q5: What software can I use to perform runs tests?
A5: Most statistical software packages (e.g., R, SPSS, SAS) provide functions for conducting runs tests.
Q6: Can runs tests be used for multivariate data?
A6: Standard runs tests are designed for univariate data. Extensions or adaptations might be necessary to handle multivariate data, often involving applying the runs test to each variable separately.
Practical Tips
-
Clearly Define Your Categories: Ensure your categories are mutually exclusive and exhaustive.
-
Consider Autocorrelation: Assess autocorrelation before conducting the test, as it can influence results.
-
Choose the Right Test: Select the runs test most appropriate for your data and research question.
-
Interpret the Results Carefully: Don't solely rely on the p-value; consider the magnitude of the deviation from the expected number of runs.
-
Use Visualizations: Create visual representations of your data sequence to aid in interpretation.
-
Combine with Other Methods: Use runs tests in conjunction with other statistical techniques for a more thorough analysis.
-
Document Your Methodology: Clearly describe your data, the chosen runs test, and your interpretation of the results.
-
Consider the Sample Size: Runs tests may have lower power with smaller sample sizes.
Final Conclusion
Runs tests offer a valuable and versatile approach to analyzing sequential data for randomness. Their simplicity, non-parametric nature, and sensitivity to patterns make them an indispensable tool across numerous disciplines. By understanding the principles, types, and applications of runs tests, researchers and analysts can gain crucial insights into the underlying structure of their data, leading to better informed decisions and a deeper understanding of complex phenomena. The power of runs tests lies not only in their statistical significance but also in their ability to reveal subtle patterns often overlooked by other methods. Further exploration of the nuances and variations of runs tests, alongside the integration with other statistical techniques, opens exciting possibilities for data analysis in diverse fields.
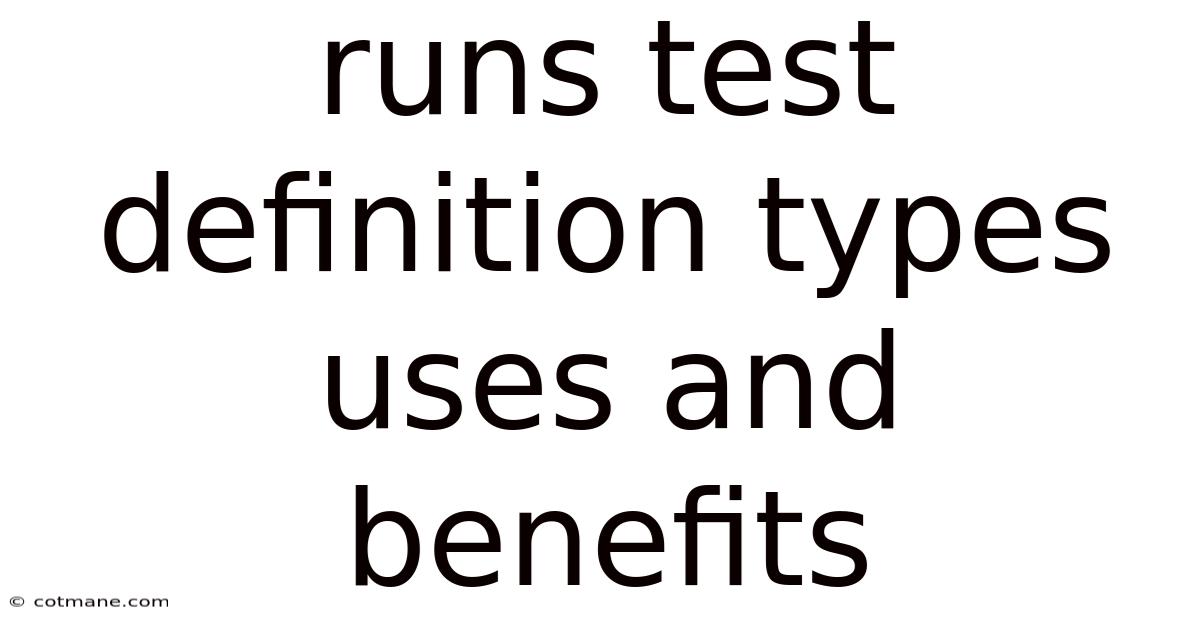
Thank you for visiting our website wich cover about Runs Test Definition Types Uses And Benefits. We hope the information provided has been useful to you. Feel free to contact us if you have any questions or need further assistance. See you next time and dont miss to bookmark.
Also read the following articles
Article Title | Date |
---|---|
Sec Form N 1a Definition | Apr 03, 2025 |
Sec Form S 4 Definition Purpose And Filing Requirements | Apr 03, 2025 |
Best Buy Credit Card Review | Apr 03, 2025 |
Sec Form N 18f 1 Definition | Apr 03, 2025 |
Schedule To T Definition | Apr 03, 2025 |