Sample Distribution Definition How Its Used With An Example
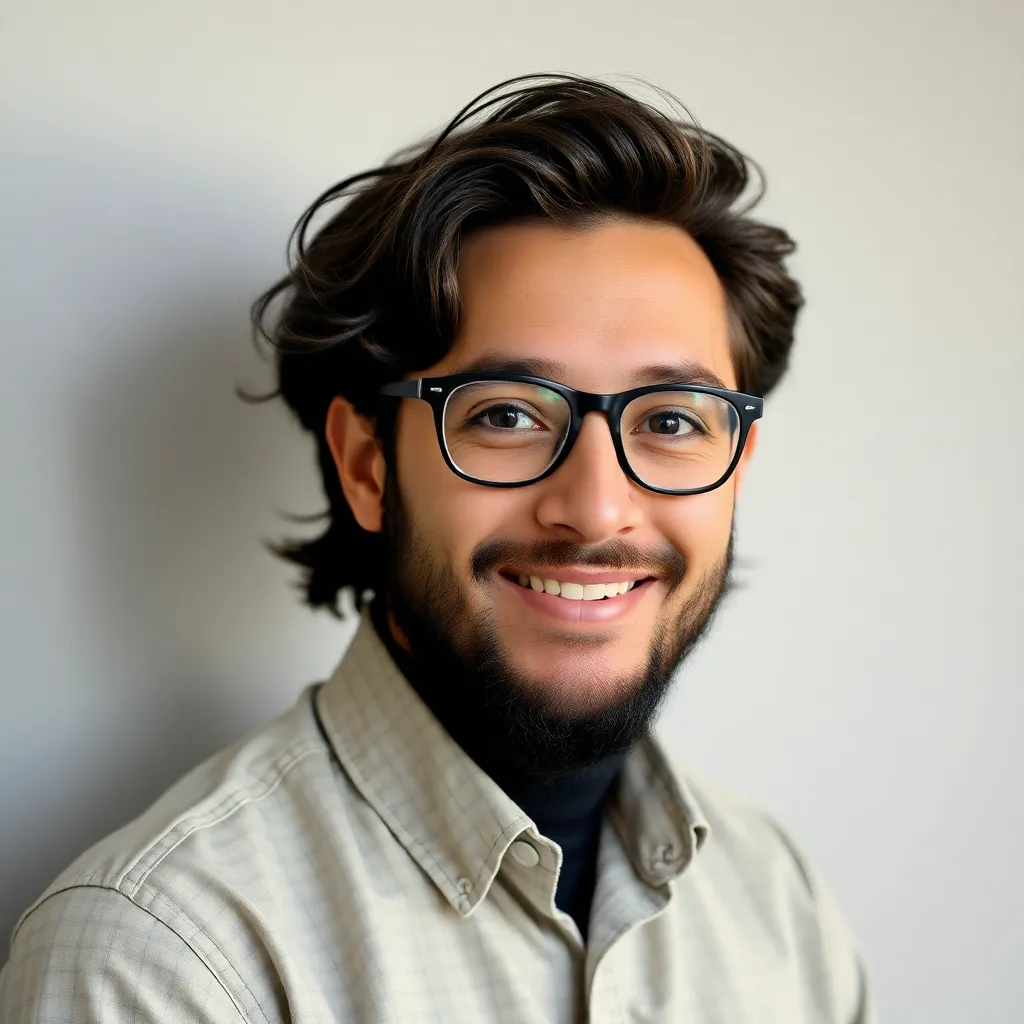
adminse
Apr 03, 2025 · 9 min read

Table of Contents
Unlocking the Power of Sample Distribution: Definition, Applications, and a Real-World Example
What makes understanding sample distribution crucial in data analysis?
Sample distribution is the cornerstone of statistical inference, allowing us to draw meaningful conclusions about populations based on limited data.
Editor’s Note: This article on sample distribution was published today, providing the most current insights and understanding of this critical statistical concept.
Why Sample Distribution Matters
In the realm of statistics, we often encounter situations where studying an entire population is impractical or impossible. Imagine trying to survey every single person in a country to understand their voting preferences – a monumental task! This is where sample distribution steps in. It allows researchers and analysts to draw inferences about a population's characteristics based on a smaller, representative sample. Understanding sample distributions is vital because it forms the basis for hypothesis testing, confidence intervals, and numerous other statistical procedures crucial in various fields like healthcare, finance, marketing, and social sciences. The accuracy and reliability of these procedures depend heavily on the proper understanding and application of sample distributions. Improper sampling techniques or misinterpretations of the sample distribution can lead to flawed conclusions and potentially costly errors. Therefore, mastering this concept is essential for anyone involved in data-driven decision-making. The ability to correctly interpret sample distributions enables better forecasting, improved risk management, and more effective resource allocation.
Overview of the Article
This article provides a comprehensive exploration of sample distribution. We will define it clearly, discuss its different types, illustrate its uses with a detailed real-world example, and examine the connection between sample distribution and population distribution. Readers will gain a practical understanding of how to interpret sample distributions and appreciate their importance in various analytical processes. The article culminates with a FAQ section and actionable tips to further enhance understanding and application of this crucial statistical concept.
Research and Effort Behind the Insights
This article draws upon established statistical principles and utilizes examples drawn from widely recognized research methodologies. The information presented is consistent with accepted statistical practices and aims to provide clarity and understanding for a broad audience.
Key Takeaways
Key Concept | Description |
---|---|
Sample Distribution Definition | The probability distribution of a statistic (e.g., sample mean, sample variance) calculated from a random sample. |
Types of Sample Distributions | Includes sampling distributions of the mean, variance, proportion, and others. |
Central Limit Theorem | States that the sampling distribution of the mean approaches a normal distribution as sample size increases. |
Applications | Hypothesis testing, confidence intervals, estimation of population parameters. |
Importance | Enables drawing inferences about populations based on sample data. |
Smooth Transition to Core Discussion
Now, let's delve into the core aspects of sample distribution, starting with its precise definition and exploring its various types.
Exploring the Key Aspects of Sample Distribution
-
Defining Sample Distribution: A sample distribution is the probability distribution of a statistic obtained from a large number of samples drawn from a population. The statistic could be the sample mean (average), sample variance (spread), sample proportion (percentage), or any other summary measure. It's crucial to remember that the sample distribution is not the distribution of the data within a single sample; it's the distribution of the statistic across many samples.
-
Types of Sample Distributions: Several types of sample distributions exist, each tailored to a specific statistic. The most common are:
- Sampling Distribution of the Mean: This is perhaps the most frequently used sample distribution. It describes the distribution of sample means calculated from numerous random samples drawn from the same population. The Central Limit Theorem plays a critical role here.
- Sampling Distribution of the Proportion: This distribution describes the probability distribution of sample proportions (e.g., the proportion of individuals in a sample who prefer a particular brand). This is particularly relevant in surveys and opinion polls.
- Sampling Distribution of the Variance: This describes the distribution of sample variances calculated from numerous random samples. It is important in assessing the variability within the population.
- Other Sampling Distributions: Other statistics, like the median, range, or even more complex statistics, can also have their corresponding sampling distributions. The choice of which sampling distribution to use depends entirely on the statistic being analyzed.
-
The Central Limit Theorem (CLT): The CLT is a fundamental theorem in statistics that underpins much of the theory behind sample distributions. It states that, regardless of the shape of the original population distribution, the sampling distribution of the mean will approximate a normal distribution as the sample size increases (generally, n ≥ 30). This is incredibly useful because it allows us to use the properties of the normal distribution to make inferences about the population mean even if we don't know the population distribution's shape.
-
Applications of Sample Distribution: The applications of sample distribution are vast and crucial to statistical inference. Here are some key uses:
- Hypothesis Testing: Sample distributions are fundamental to hypothesis testing. We use them to determine the probability of observing a particular sample statistic if the null hypothesis is true.
- Confidence Intervals: Sample distributions are also crucial in constructing confidence intervals, which provide a range of plausible values for a population parameter (e.g., the population mean) based on sample data.
- Estimation of Population Parameters: Sample distributions help us estimate population parameters, providing insights into the population's characteristics based on sample data.
Closing Insights
Understanding sample distributions is paramount for accurate and reliable statistical analysis. It forms the bridge between sample data and inferences about the population, enabling robust decision-making across numerous fields. The Central Limit Theorem's power simplifies many analytical processes, allowing us to leverage the well-understood properties of the normal distribution to draw conclusions about populations. By understanding the different types of sample distributions and their applications, analysts can effectively analyze data, test hypotheses, and build confidence intervals to support data-driven decisions. The accurate interpretation and utilization of sample distributions contribute directly to the reliability and validity of research findings.
Exploring the Connection Between Standard Error and Sample Distribution
The standard error is intrinsically linked to the sample distribution. It measures the variability of the sampling distribution of a statistic, specifically the sample mean. A smaller standard error indicates that the sample means from multiple samples are clustered closely around the population mean, suggesting a more precise estimate. Conversely, a larger standard error implies greater variability in the sample means, leading to less precise estimates. The standard error is inversely proportional to the square root of the sample size; larger sample sizes generally lead to smaller standard errors and thus more precise estimations.
Further Analysis of Standard Error
The standard error's significance lies in its role in hypothesis testing and confidence interval construction. In hypothesis testing, the standard error is used to calculate the test statistic, which determines whether to reject or fail to reject the null hypothesis. In constructing confidence intervals, the standard error helps define the margin of error, indicating the range within which the true population parameter is likely to lie.
Factor Affecting Standard Error | Effect |
---|---|
Sample Size (n) | Inversely proportional (larger n, smaller SE) |
Population Standard Deviation | Directly proportional (larger σ, larger SE) |
FAQ Section
-
Q: What is the difference between a sample distribution and a population distribution? A: A population distribution describes the probability distribution of all values in a population, while a sample distribution describes the probability distribution of a statistic (like the mean) calculated from multiple samples drawn from that population.
-
Q: Why is the Central Limit Theorem so important? A: The CLT simplifies statistical analysis by stating that the sampling distribution of the mean will approximate a normal distribution regardless of the population distribution's shape, as long as the sample size is sufficiently large.
-
Q: How does sample size affect the sample distribution? A: Larger sample sizes lead to sample distributions that are more closely centered around the population parameter and have smaller standard errors, resulting in more precise estimations.
-
Q: What are some common mistakes in interpreting sample distributions? A: Common mistakes include misinterpreting a sample distribution as the population distribution, ignoring the sample size's effect on the distribution, and incorrectly applying the Central Limit Theorem.
-
Q: Can sample distributions be used for non-numerical data? A: While the CLT primarily applies to numerical data, similar concepts apply to categorical data using the sampling distribution of proportions.
-
Q: How can I improve the accuracy of my sample distribution? A: Ensure your sampling method is random and representative of the population, increase your sample size, and consider the characteristics of your data when choosing the appropriate sampling distribution.
Practical Tips
-
Use Random Sampling: Employ random sampling techniques to ensure your sample is representative of the population.
-
Increase Sample Size: Larger samples generally lead to more accurate estimations and more reliable sample distributions.
-
Check for Normality: Verify if your sample distribution approximates a normal distribution, especially when using procedures that rely on this assumption (e.g., t-tests).
-
Understand Standard Error: Calculate and interpret the standard error to understand the precision of your estimates.
-
Consider the Population Distribution: If the population distribution is known or can be estimated, incorporate this knowledge into your analysis.
-
Use Appropriate Statistical Software: Statistical software packages can significantly aid in calculating sample statistics and visualizing sample distributions.
-
Consult with a Statistician: For complex analyses or when dealing with unusual data, consulting a statistician is advisable to ensure the correct application of sample distribution concepts.
-
Document Your Methodology: Carefully document your sampling methods, data analysis, and interpretations to ensure transparency and reproducibility.
Final Conclusion
Sample distribution is a cornerstone of statistical inference, enabling us to draw meaningful conclusions about populations based on sample data. Understanding its principles, different types, and applications is essential for anyone working with data. The Central Limit Theorem simplifies many analytical procedures, while the standard error provides a crucial measure of the precision of our estimations. By following best practices in sampling, analysis, and interpretation, we can leverage the power of sample distribution to derive accurate and reliable insights, leading to more informed and effective decision-making. Continuous learning and practical application are key to mastering this critical statistical concept and its profound implications for various fields.
Latest Posts
Related Post
Thank you for visiting our website which covers about Sample Distribution Definition How Its Used With An Example . We hope the information provided has been useful to you. Feel free to contact us if you have any questions or need further assistance. See you next time and don't miss to bookmark.