Sample Selection Bias Definition Examples And How To Avoid
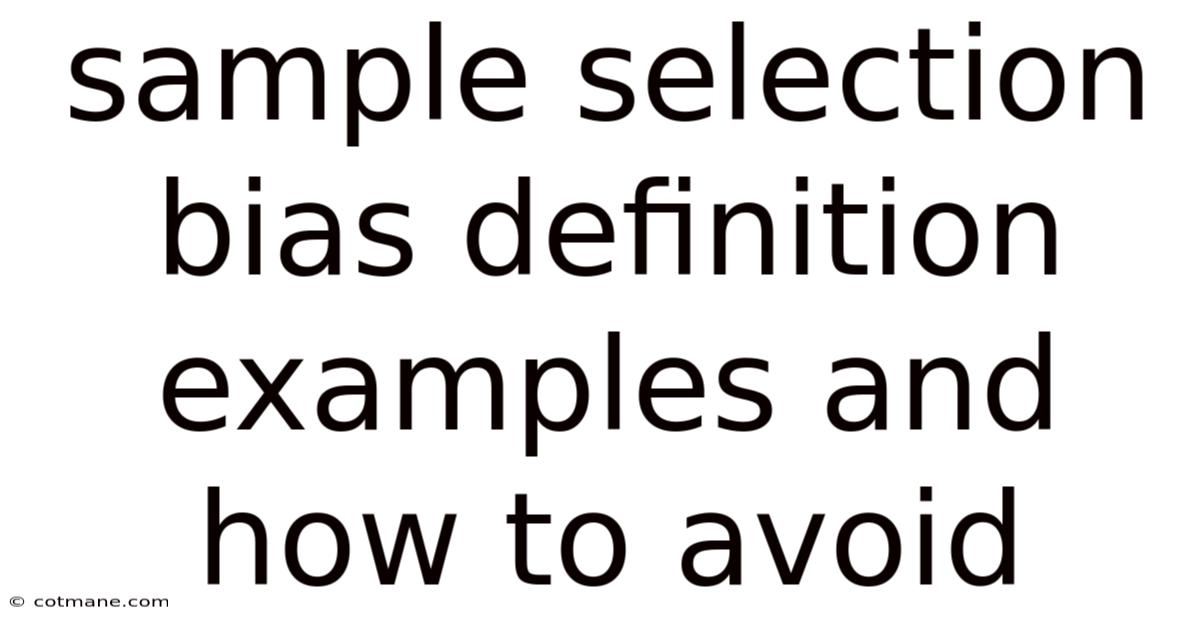
Discover more detailed and exciting information on our website. Click the link below to start your adventure: Visit Best Website meltwatermedia.ca. Don't miss out!
Table of Contents
Sample Selection Bias: Definition, Examples, and Mitigation Strategies
What makes sample selection bias a critical issue in research and data analysis?
Sample selection bias can invalidate research findings, leading to inaccurate conclusions and flawed decision-making across various fields.
Editor's Note: This article on sample selection bias has been published today, providing up-to-date insights and practical strategies for researchers and data analysts.
Why Sample Selection Bias Matters
Sample selection bias, a pervasive threat to research validity, occurs when the sample chosen for a study doesn't accurately represent the population it intends to describe. This skewed representation can drastically distort results, leading to erroneous conclusions and ineffective strategies. The implications are far-reaching, impacting fields from medical research and public policy to marketing and social science. A biased sample can lead to:
- Inaccurate estimations: Statistics derived from a biased sample will poorly estimate the true population parameters. This can lead to incorrect predictions, forecasts, and policy recommendations.
- Misleading conclusions: Studies affected by selection bias may reach conclusions that contradict reality, potentially harming individuals, organizations, or entire communities. For example, a biased clinical trial might lead to the approval of an ineffective or even harmful treatment.
- Wasted resources: Research projects based on flawed sampling methods waste time, money, and effort. The resulting inaccurate findings might necessitate costly revisions and repeated studies.
- Erosion of public trust: When flawed research reaches the public domain, it can erode trust in scientific findings and evidence-based decision-making.
Understanding and mitigating sample selection bias is crucial for ensuring the reliability and validity of research and data analysis. This requires careful consideration of sampling methods, rigorous data collection protocols, and robust statistical techniques.
Overview of the Article
This article will delve into the intricacies of sample selection bias. We will explore its definition, dissect various examples across different domains, and detail practical strategies to avoid or mitigate its effects. Readers will gain a comprehensive understanding of this critical issue, equipping them with the knowledge and tools to design and conduct more robust and reliable research.
Research and Effort Behind the Insights
The information presented in this article is derived from extensive research, drawing upon established statistical literature, published case studies, and best practices from various disciplines. The analysis synthesizes key concepts and provides practical examples to enhance understanding and application.
Key Takeaways
Key Aspect | Description |
---|---|
Definition of Sample Selection Bias | A systematic error that arises when the sample used for a study does not accurately represent the population from which it was drawn. |
Types of Sample Selection Bias | Includes volunteer bias, convenience sampling bias, healthy worker effect, and more. |
Consequences of Selection Bias | Inaccurate estimates, misleading conclusions, wasted resources, and eroded public trust. |
Mitigation Strategies | Employing probability sampling techniques, careful consideration of inclusion/exclusion criteria, adjusting for bias statistically, and using multiple data sources. |
Smooth Transition to Core Discussion
Let's now explore the key aspects of sample selection bias in greater detail, beginning with its fundamental definition and moving onto various examples and effective mitigation techniques.
Exploring the Key Aspects of Sample Selection Bias
-
Defining Sample Selection Bias: Sample selection bias, also known as ascertainment bias, occurs when the method used to select participants for a study systematically favors certain characteristics, resulting in a sample that is not representative of the larger population. This non-random selection introduces a systematic error that can distort the study’s results.
-
Types of Sample Selection Bias: Several forms of sample selection bias exist, each with its unique mechanisms. Some prominent types include:
-
Volunteer Bias: This occurs when individuals who volunteer to participate in a study differ systematically from those who do not. Volunteers might be more motivated, have specific characteristics (e.g., higher education levels), or hold stronger opinions than the general population.
-
Convenience Sampling Bias: This bias arises when researchers select participants based on ease of access rather than representativeness. This might involve using readily available samples like university students or employees of a particular company, which may not reflect the characteristics of the broader population.
-
Healthy Worker Effect: This occurs in occupational health studies where employed individuals tend to be healthier than the general population. Because they are working, they might have better health habits or pre-existing health conditions that prevent them from working might be excluded.
-
Referral Bias: This happens when participants are referred to a study by other participants, creating a non-random sampling mechanism and potentially skewing the sample towards certain characteristics shared among the referral network.
-
Length-Time Bias: In studies observing the occurrence of events over time, length-time bias refers to a situation where long-latency diseases are more likely to be detected than diseases with shorter latency periods, leading to an overestimation or underestimation of risk factors.
-
-
Consequences of Sample Selection Bias: The implications of sample selection bias can be severe. Biased samples can lead to inaccurate estimations of population parameters, misleading conclusions, wasted resources, and a diminished level of public trust in research.
-
Mitigating Sample Selection Bias: Avoiding or mitigating sample selection bias requires a proactive approach during all stages of research:
-
Probability Sampling Techniques: Employing probability sampling methods (simple random sampling, stratified random sampling, cluster sampling) ensures that every member of the population has a known, non-zero probability of being selected, minimizing selection bias.
-
Defining Inclusion/Exclusion Criteria: Explicitly defining criteria for participant inclusion and exclusion helps to standardize the selection process and minimize researcher bias. These criteria should be based on the research question and the characteristics relevant to the study, not on convenience.
-
Stratification: If the population is heterogeneous, stratifying the sample based on relevant characteristics (e.g., age, gender, ethnicity) ensures representation of different subgroups within the sample.
-
Statistical Adjustments: While preventing bias is ideal, statistical techniques like weighting or inverse probability weighting can sometimes adjust for known selection biases in the analysis phase. However, these methods depend on having sufficient information on the selection mechanism.
-
Using Multiple Data Sources: Triangulation of data from multiple sources (surveys, administrative data, observational studies) can help to corroborate findings and identify potential biases.
-
Randomization: In experimental studies, random assignment of participants to treatment and control groups is crucial for minimizing selection bias.
-
-
Real-World Examples:
-
A pharmaceutical company conducts a clinical trial for a new drug using only patients from a single hospital. This might result in a biased sample if the hospital's patient population differs systematically from the broader population.
-
A survey on political opinions is conducted only through online platforms. This might exclude individuals without internet access, leading to a biased sample that does not represent the views of the entire population.
-
A study on the effectiveness of a new teaching method uses only volunteer teachers. These teachers might be more enthusiastic and dedicated, leading to biased results that don't reflect the effectiveness of the method in a typical classroom setting.
-
Exploring the Connection Between Non-response Bias and Sample Selection Bias
Non-response bias, a closely related concept, occurs when a significant portion of selected participants fail to respond to a survey or study. This non-response can introduce bias if the characteristics of non-respondents differ systematically from respondents. For example, individuals with strong opinions might be more likely to respond to a survey, while those with neutral opinions might be less likely. This is a form of selection bias because the final sample differs systematically from the initially selected sample. Mitigation strategies include incentivizing participation, employing multiple contact methods, and statistically adjusting for non-response.
Further Analysis of Non-response Bias
Factor Influencing Non-response Bias | Effect | Mitigation Strategy |
---|---|---|
Length of questionnaire | Longer questionnaires lead to higher non-response rates. | Shorten questionnaire, use multiple short questionnaires |
Complexity of questions | Difficult questions increase non-response. | Simplify questions, pre-test questionnaire |
Mode of administration | Mode (e.g., mail, phone, online) affects response rates. | Employ multiple modes, optimize for target population |
Respondent characteristics | Demographics, attitudes, and beliefs can influence participation. | Adjust sampling weights to account for differences. |
Timing of data collection | Season, time of day affect response rates. | Optimize timing for maximal response. |
FAQ Section
-
Q: What is the difference between sampling error and sample selection bias? A: Sampling error is the inherent variability that arises from using a sample to estimate population parameters. It's a random error. Sample selection bias, on the other hand, is a systematic error due to a non-representative sample.
-
Q: Can sample selection bias be completely avoided? A: It's extremely difficult to completely eliminate sample selection bias, but careful planning and employing appropriate sampling techniques can significantly reduce its impact.
-
Q: How can I detect sample selection bias in published research? A: Look for detailed descriptions of the sampling method, the characteristics of the sample compared to the population, and any discussion of potential biases.
-
Q: Is it always necessary to use probability sampling? A: Probability sampling is ideal for minimizing bias and making inferences to the population. However, in some cases, non-probability sampling might be used (e.g., qualitative research), but its limitations must be acknowledged.
-
Q: What is the role of power analysis in minimizing sample selection bias? A: Power analysis helps determine the appropriate sample size to detect a statistically significant effect. While it doesn't directly address selection bias, a sufficiently large sample size can sometimes mitigate the impact of minor biases.
-
Q: How can I communicate the limitations due to potential sample selection bias in my research? A: Transparently discuss the limitations of your sampling methodology in your research report. Acknowledge potential biases and their implications for your results.
Practical Tips to Avoid Sample Selection Bias
-
Define your target population precisely: Clearly specify the population you are studying to avoid inadvertently excluding or including irrelevant groups.
-
Choose an appropriate sampling method: Select a probability sampling method that best suits your research question and resources.
-
Develop clear inclusion/exclusion criteria: Establish objective criteria for participant selection to avoid researcher bias.
-
Maximize response rates: Employ strategies to increase response rates in surveys or experiments, minimizing non-response bias.
-
Stratify your sample: If relevant subgroups exist within the population, stratify your sample to ensure adequate representation.
-
Pilot test your methodology: Conduct a small-scale pilot study to identify and address potential issues before launching the main study.
-
Employ statistical adjustments: If bias is detected, explore statistical methods (weighting, imputation) to adjust for it.
-
Use multiple data sources: If possible, gather data from various sources to corroborate findings and identify inconsistencies.
Final Conclusion
Sample selection bias is a significant threat to the validity of research findings. However, through careful planning, meticulous methodology, and a thorough understanding of potential sources of bias, researchers can significantly minimize its impact. By employing appropriate sampling techniques, defining clear inclusion/exclusion criteria, maximizing response rates, and utilizing statistical adjustments where necessary, researchers can enhance the reliability and generalizability of their studies, ultimately contributing to a more robust and credible body of knowledge. The pursuit of unbiased research requires ongoing vigilance and a commitment to rigorous methodologies throughout the entire research process. Remember, the goal is not perfect representation, but rather a systematic reduction of bias that allows for more accurate and reliable inferences about the population of interest.
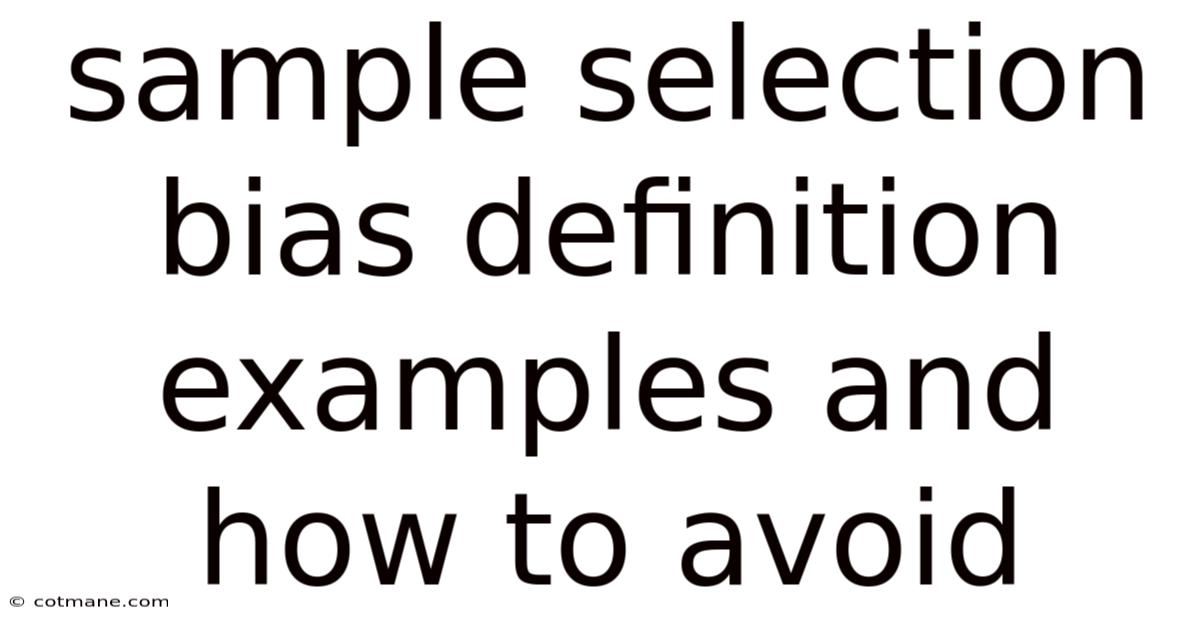
Thank you for visiting our website wich cover about Sample Selection Bias Definition Examples And How To Avoid. We hope the information provided has been useful to you. Feel free to contact us if you have any questions or need further assistance. See you next time and dont miss to bookmark.
Also read the following articles
Article Title | Date |
---|---|
Sec Form F 8 Definition | Apr 03, 2025 |
Rural Housing Service Rhs Definition | Apr 03, 2025 |
Sec Form Dfan14a Definition | Apr 03, 2025 |
What Percentage Of Personal Finance Is Behavior | Apr 03, 2025 |
Semi Strong Form Efficiency Definition And Market Hypothesis | Apr 03, 2025 |