Sample Size Neglect Defined
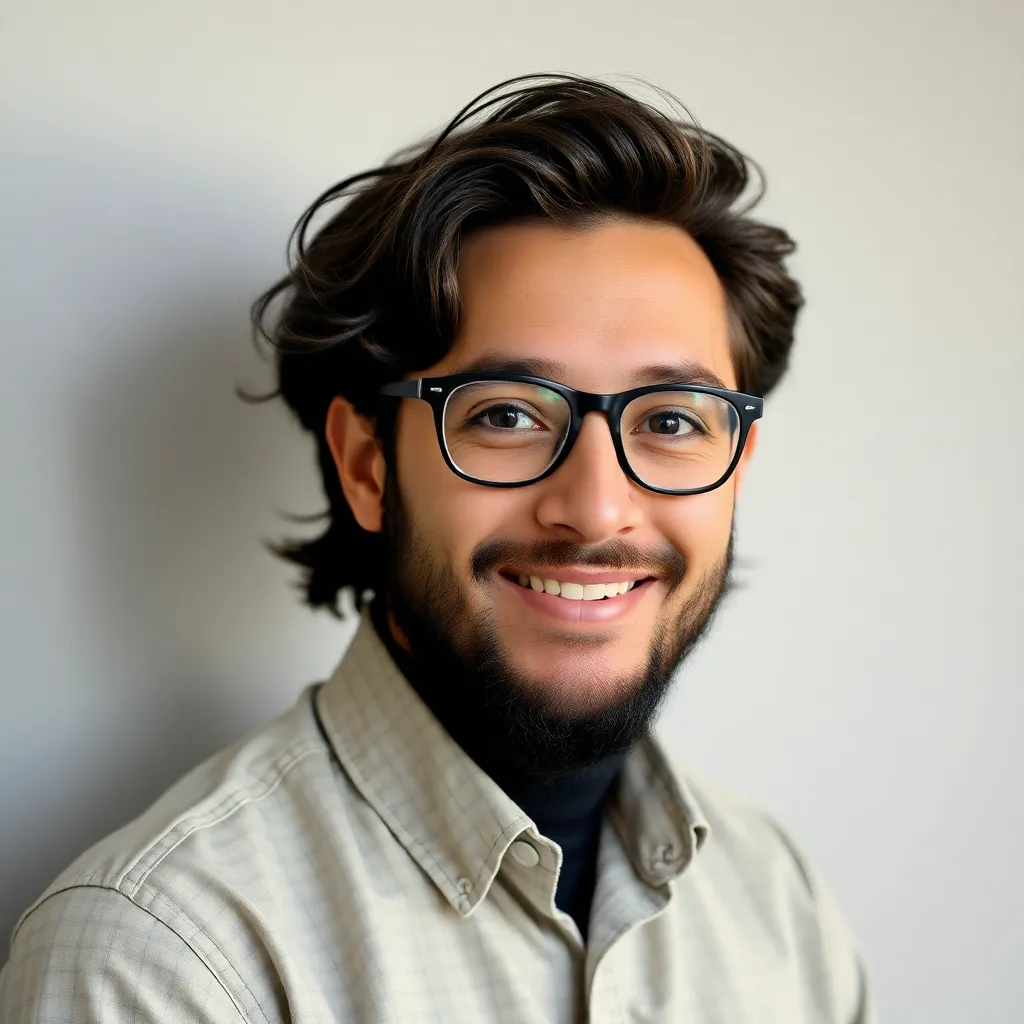
adminse
Apr 03, 2025 · 9 min read

Table of Contents
Unveiling the Bias: A Deep Dive into Sample Size Neglect
What makes sample size neglect a significant cognitive bias in today’s data-driven world?
Sample size neglect is a pervasive cognitive bias that significantly impacts our ability to interpret data accurately, leading to flawed conclusions and suboptimal decisions across various fields.
Editor’s Note: Sample size neglect has been published today. This article provides a comprehensive overview of this critical cognitive bias, its manifestations, and strategies for mitigation.
Why Sample Size Neglect Matters
In our increasingly data-driven world, the ability to interpret statistical information accurately is paramount. From medical research and financial markets to social sciences and everyday decision-making, our reliance on data analysis is undeniable. However, a common pitfall that undermines the validity of these analyses is sample size neglect – the tendency to overgeneralize from small samples, disregarding the inherent uncertainty associated with limited data. This bias can lead to erroneous conclusions, flawed predictions, and ultimately, poor decision-making. Understanding and mitigating sample size neglect is crucial for navigating the complexities of data interpretation and ensuring the reliability of our conclusions. Its effects ripple through various domains, impacting everything from public health initiatives based on small clinical trials to market analyses skewed by limited customer feedback. The cost of this neglect can range from minor inconveniences to significant financial losses and even public health crises.
Overview of the Article
This article explores the key aspects of sample size neglect, its cognitive underpinnings, and its pervasive influence across various disciplines. Readers will gain a deeper understanding of this bias, its mechanisms, and practical strategies to avoid its pitfalls. We will examine real-world examples, discuss the statistical principles underpinning its impact, and provide actionable advice for interpreting data more accurately. The goal is to equip readers with the tools and knowledge needed to make sound judgments based on data, mitigating the risks associated with sample size neglect.
Research and Effort Behind the Insights
This article draws upon extensive research in cognitive psychology, behavioral economics, and statistics. It integrates findings from numerous peer-reviewed studies, analyzing the prevalence and consequences of sample size neglect across diverse contexts. The analysis incorporates statistical principles, illustrative examples, and practical recommendations to foster a comprehensive understanding of this crucial bias. The information presented is supported by empirical evidence and aims to provide a rigorous and insightful exploration of sample size neglect.
Key Takeaways
Key Insight | Explanation |
---|---|
Small samples are inherently unreliable. | Limited data increases the likelihood of sampling error, leading to inaccurate generalizations. |
Larger samples yield more reliable results. | Increased sample size reduces sampling error and improves the accuracy of estimates and inferences. |
Intuition often misleads regarding sample size. | Our cognitive biases lead us to overestimate the reliability of small samples, even when statistical principles dictate otherwise. |
Awareness is the first step towards mitigation. | Recognizing the bias is crucial for actively engaging in strategies to counteract its influence on data interpretation and decision-making. |
Statistical literacy is essential. | Understanding basic statistical concepts, such as confidence intervals and margin of error, helps in evaluating the reliability of data. |
Smooth Transition to Core Discussion
Let’s delve deeper into the key aspects of sample size neglect, starting with its cognitive roots and progressing to its practical implications and mitigation strategies.
Exploring the Key Aspects of Sample Size Neglect
-
The Cognitive Roots of Neglect: Sample size neglect stems from several cognitive biases, including the availability heuristic (overestimating the likelihood of events easily recalled), representativeness heuristic (judging probability based on similarity to a prototype), and confirmation bias (favoring information confirming pre-existing beliefs). These biases interfere with our ability to accurately assess the impact of sample size on the reliability of data.
-
Manifestations Across Domains: Sample size neglect manifests across a multitude of fields. In medical research, small clinical trials can lead to the overestimation of treatment efficacy. In finance, investment decisions based on limited market data can result in significant losses. In social sciences, generalizations about population trends from small surveys can perpetuate stereotypes and misinformation.
-
The Role of Probability and Statistics: Understanding basic probability and statistical concepts is crucial for mitigating sample size neglect. Concepts like standard error, confidence intervals, and p-values provide a quantitative measure of uncertainty associated with sample data. A larger sample size reduces the standard error, resulting in narrower confidence intervals and a more precise estimate of the population parameter.
-
Visual Representations and Misinterpretations: Visual representations of data, such as graphs and charts, can be particularly susceptible to misinterpretations due to sample size neglect. Small sample sizes can lead to misleading visual impressions, exaggerating trends or differences that may not be statistically significant.
-
Strategies for Mitigation: Several strategies can help mitigate sample size neglect. These include employing rigorous statistical methods, seeking independent verification of results, and focusing on effect sizes rather than just statistical significance. Also, actively seeking out and critically evaluating multiple studies helps counter the influence of any single small-scale study.
Closing Insights
Sample size neglect is not merely a statistical anomaly; it's a deeply ingrained cognitive bias with far-reaching consequences. Its impact extends across various sectors, influencing decisions with significant implications. Recognizing this bias, understanding its underlying mechanisms, and employing rigorous analytical strategies are essential for accurate data interpretation and sound decision-making. By embracing a critical and statistically informed approach to data analysis, individuals and organizations can significantly reduce the risks associated with this pervasive cognitive bias. This includes understanding the limitations of small samples and seeking out larger, more representative datasets whenever possible. Furthermore, promoting statistical literacy and critical thinking skills will greatly improve our collective ability to interpret data effectively and avoid the pitfalls of sample size neglect.
Exploring the Connection Between Confirmation Bias and Sample Size Neglect
Confirmation bias, the tendency to favor information confirming existing beliefs, exacerbates the effects of sample size neglect. Individuals may selectively focus on small samples that support their preconceived notions, disregarding larger datasets or contradictory evidence. This interplay can lead to entrenched beliefs even in the face of overwhelming statistical evidence to the contrary. For example, a researcher might selectively analyze a small subset of data supporting a hypothesis, overlooking a larger dataset that contradicts it, significantly underestimating the overall uncertainty and the need for a larger sample size. The role of confirmation bias is further amplified by the availability heuristic. Small, memorable cases are more readily available in memory and can disproportionately influence judgments, even when statistically less representative of the population.
Further Analysis of Confirmation Bias
Confirmation bias operates through several mechanisms. It influences what information is actively sought, how information is interpreted, and how it is remembered. Individuals often exhibit motivated reasoning, interpreting ambiguous data in a way that confirms pre-existing beliefs. This can manifest in the selective search for evidence, the biased interpretation of data, and the selective recall of information. The table below illustrates how confirmation bias interacts with sample size neglect in different contexts:
Context | Confirmation Bias Manifestation | Sample Size Neglect Manifestation | Combined Effect |
---|---|---|---|
Medical Research | Focusing on studies supporting a preferred treatment. | Overestimating the efficacy of a treatment based on a small trial. | Incorrect conclusions about treatment effectiveness. |
Investment Decisions | Seeking information validating a specific investment strategy. | Basing investment decisions on limited market data. | Poor investment outcomes due to inaccurate market assessment. |
Social Science | Emphasizing data confirming pre-existing societal stereotypes. | Generalizing about population trends based on small surveys. | Reinforcing inaccurate societal stereotypes and biases. |
FAQ Section
-
Q: What is the minimum acceptable sample size? A: There's no universal minimum. The required sample size depends on the desired level of precision, the variability in the population, and the statistical test being used. Power analysis is often used to determine the appropriate sample size.
-
Q: How can I avoid sample size neglect in my own research? A: Employ rigorous statistical methods, conduct power analysis before data collection, and ensure your sample is representative of the population of interest. Transparency in methodology and data reporting is essential.
-
Q: Is it always wrong to use small samples? A: Not necessarily. Pilot studies with small samples can be valuable for preliminary investigations or exploratory research. However, conclusions drawn from small samples should be treated cautiously and not generalized to larger populations without further confirmation.
-
Q: How do confidence intervals help address sample size neglect? A: Confidence intervals provide a range of values within which the true population parameter is likely to fall, reflecting the uncertainty due to sampling error. Wider intervals associated with small samples highlight the limitations of the data.
-
Q: Why does sample size matter more for some studies than others? A: The impact of sample size is influenced by the variability of the phenomenon being studied and the effect size of interest. Highly variable phenomena or studies seeking to detect small effects require larger sample sizes.
-
Q: How can I tell if a study is vulnerable to sample size neglect? A: Look for studies with small sample sizes, limited descriptions of the sampling method, and a lack of statistical measures reflecting uncertainty (e.g., confidence intervals, p-values). Caution is warranted if the study makes strong conclusions based on limited data.
Practical Tips
-
Understand statistical power: Before collecting data, perform a power analysis to determine the sample size needed to detect a meaningful effect.
-
Use appropriate statistical methods: Choose statistical tests that are robust and account for the uncertainty associated with sampling error.
-
Report confidence intervals: Always report confidence intervals along with point estimates to communicate the uncertainty associated with the results.
-
Consider effect sizes: Focus on the magnitude of the effect rather than just statistical significance. A small effect might be statistically significant with a large sample but not practically relevant.
-
Replicate studies: Look for studies that have been replicated with larger samples to increase confidence in their findings.
-
Be skeptical of anecdotal evidence: Avoid relying on isolated cases or anecdotes as evidence for broader claims.
-
Visualize data carefully: Use appropriate visual representations to avoid misleading impressions due to small sample sizes.
-
Consult with a statistician: For complex analyses or when uncertainty about sample size is high, consult with a statistician to ensure appropriate methodology.
Final Conclusion
Sample size neglect represents a significant challenge in interpreting data accurately. Its insidious nature lies in its ability to subtly distort our understanding, leading to flawed conclusions and potentially harmful decisions. By fostering a greater awareness of this bias, emphasizing statistical literacy, and employing rigorous analytical techniques, we can significantly mitigate its effects and improve the reliability of inferences drawn from data. The journey towards data-driven decision-making necessitates a commitment to understanding the limitations of data, acknowledging the role of cognitive biases, and embracing a critical, statistically informed approach. Only then can we truly harness the power of data while avoiding the pitfalls of sample size neglect.
Latest Posts
Related Post
Thank you for visiting our website which covers about Sample Size Neglect Defined . We hope the information provided has been useful to you. Feel free to contact us if you have any questions or need further assistance. See you next time and don't miss to bookmark.