Sampling Errors In Statistics Definition Types And Calculation
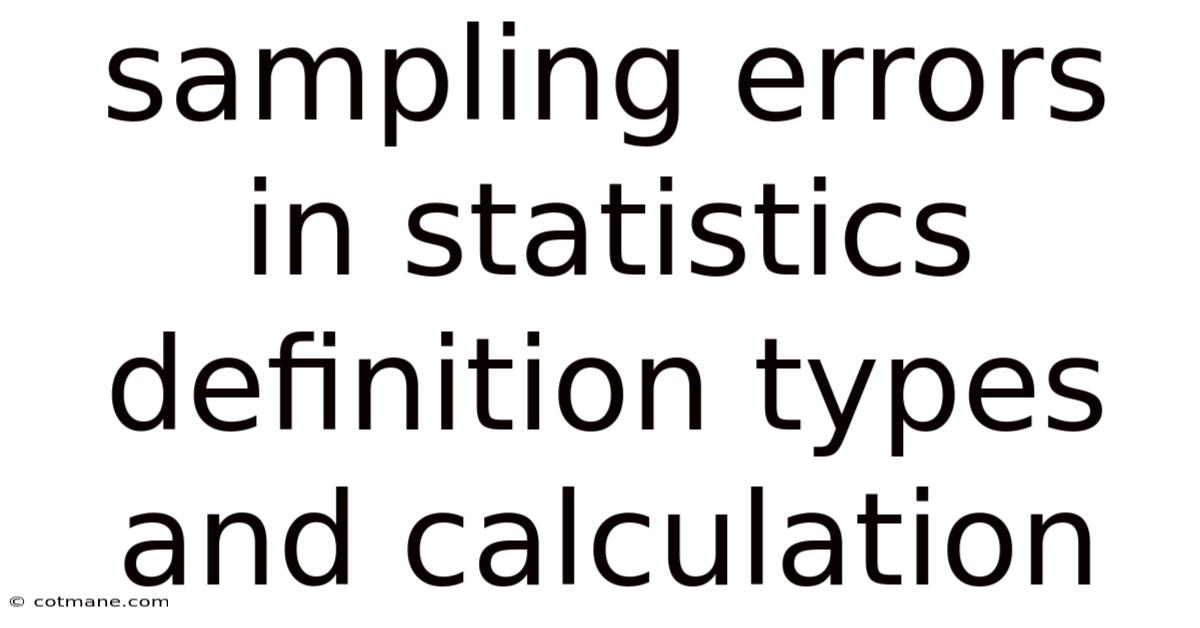
Discover more detailed and exciting information on our website. Click the link below to start your adventure: Visit Best Website meltwatermedia.ca. Don't miss out!
Table of Contents
Sampling Errors in Statistics: Definition, Types, and Calculation
What makes understanding sampling errors crucial in statistical analysis?
Sampling errors are inevitable in statistical research, but understanding their nature and impact is paramount for accurate and reliable conclusions.
Editor’s Note: This comprehensive guide to sampling errors in statistics has been published today.
Why Understanding Sampling Errors Matters
In the realm of statistics, drawing conclusions about a population based on a sample is commonplace. However, this inherently introduces the possibility of error – sampling error. Understanding sampling error is not merely an academic exercise; it's fundamental to the validity and reliability of any statistical inference. Ignoring sampling error can lead to misleading conclusions, flawed decision-making, and wasted resources. Across diverse fields – from market research and public health to scientific experiments and political polling – accurate estimation and inference hinge on comprehending and mitigating the impact of sampling errors. Failing to account for them can result in skewed interpretations of data, impacting policy decisions, product development strategies, and scientific advancements. Therefore, understanding the sources, types, and methods for calculating and minimizing sampling errors is crucial for anyone working with statistical data.
Overview of the Article
This article provides a thorough exploration of sampling errors, encompassing their definition, various types, and calculation methods. Readers will gain a comprehensive understanding of how sampling errors arise, their potential impact on research findings, and strategies to minimize their effect. We will delve into the nuances of different sampling techniques and their influence on the magnitude of sampling error. The ultimate goal is to equip readers with the knowledge to critically evaluate statistical studies and draw more accurate and reliable conclusions from sample data.
Research and Effort Behind the Insights
This article draws upon a wide range of statistical literature, including textbooks, research papers, and online resources. The information presented reflects established statistical principles and methodologies. Extensive analysis of different sampling techniques and error calculation methods has been conducted to ensure accuracy and clarity. The aim is to provide a clear, concise, and readily understandable guide to this vital aspect of statistical analysis.
Key Takeaways
Key Aspect | Description |
---|---|
Definition of Sampling Error | The difference between a sample statistic and the corresponding population parameter. |
Types of Sampling Errors | Random and non-random (systematic) errors. |
Calculation of Sampling Error | Involves calculating the standard error of the mean, standard error of the proportion, or confidence intervals. |
Minimizing Sampling Error | Employing appropriate sampling techniques (e.g., random sampling, stratified sampling), increasing sample size. |
Impact of Sample Size | Larger samples generally lead to smaller sampling errors. |
Confidence Intervals | Provide a range of values within which the true population parameter likely lies. |
Smooth Transition to Core Discussion
Let's delve into the core concepts of sampling error, starting with a precise definition and exploring the different types encountered in statistical analysis.
Exploring the Key Aspects of Sampling Errors
-
Defining Sampling Error: Sampling error is the inherent discrepancy between a sample statistic (e.g., sample mean, sample proportion) calculated from a randomly selected subset of a population and the true population parameter (e.g., population mean, population proportion). This error arises simply because a sample, by its nature, cannot perfectly represent the entire population. It's a natural consequence of using a subset of the data rather than the entire dataset.
-
Types of Sampling Errors: Sampling errors are broadly classified into two main categories:
-
Random Sampling Error: This type of error is purely due to chance. It occurs because a sample, even if randomly selected, will rarely be a perfect miniature of the population. Random sampling error is unpredictable and inherent in the sampling process. It decreases as the sample size increases.
-
Non-Random or Systematic Sampling Error (Bias): This arises from flaws in the sampling method itself, leading to a consistent over- or underestimation of the population parameter. Systematic errors are not due to chance but result from biased selection procedures. Examples include selection bias (certain groups are over- or underrepresented), response bias (participants respond in a non-truthful manner), and measurement bias (errors in the measurement instrument).
-
-
Calculating Sampling Error: The precise method for calculating sampling error depends on the statistic being considered. For the mean, the standard error of the mean (SEM) is commonly used:
-
Standard Error of the Mean (SEM): SEM = σ/√n, where σ is the population standard deviation and n is the sample size. If the population standard deviation is unknown (which is usually the case), the sample standard deviation (s) is used as an estimate. Thus, SEM ≈ s/√n. The SEM quantifies the variability of the sample means around the true population mean. A smaller SEM indicates less variability and greater precision.
-
Standard Error of the Proportion: For proportions, the standard error of the proportion (SEP) is used: SEP = √[p(1-p)/n], where p is the sample proportion and n is the sample size. This measures the variability of sample proportions around the true population proportion.
-
Confidence Intervals: Confidence intervals provide a range of values within which the true population parameter is likely to fall, with a specified level of confidence (e.g., 95%). They are constructed using the sample statistic, its standard error, and the critical value from the appropriate probability distribution (often the Z-distribution or t-distribution).
-
-
Minimizing Sampling Error: Several strategies can be employed to minimize sampling error:
-
Increase Sample Size: A larger sample size generally leads to a smaller sampling error, providing a more accurate representation of the population.
-
Use Appropriate Sampling Techniques: Employing proper sampling methods, such as simple random sampling, stratified random sampling (ensuring representation from different subgroups), cluster sampling, or systematic sampling (selecting every kth element), can significantly reduce bias and improve the accuracy of estimates.
-
Careful Design and Implementation: Meticulous attention to the design and execution of the sampling process is crucial. This includes clear definition of the population, careful selection of sampling units, minimizing non-response bias, and ensuring accurate data collection.
-
Pilot Studies: Conducting a smaller pilot study before the main research can help identify and address potential problems in the sampling method or data collection process.
-
Closing Insights
Sampling error is an inherent part of statistical inference. While it cannot be entirely eliminated, its impact can be significantly minimized through careful planning, appropriate sampling techniques, and a sufficiently large sample size. Understanding the nature and magnitude of sampling error is essential for interpreting statistical results accurately and drawing reliable conclusions. Failure to account for sampling error can lead to misinterpretations, flawed decisions, and ineffective resource allocation. By employing the strategies outlined in this article, researchers and analysts can enhance the validity and reliability of their statistical findings.
Exploring the Connection Between Sample Size and Sampling Error
The relationship between sample size and sampling error is inversely proportional. As the sample size increases, the sampling error tends to decrease. This is because a larger sample provides a more representative snapshot of the population, reducing the impact of random fluctuations. The standard error of the mean (SEM) and the standard error of the proportion (SEP), both inversely related to the square root of the sample size (√n), explicitly demonstrate this relationship. For instance, quadrupling the sample size will only halve the standard error. This highlights the diminishing returns of increasing sample size beyond a certain point. While larger samples generally improve accuracy, the cost and effort involved in collecting data must also be considered.
Further Analysis of Sample Size Determination
Determining the appropriate sample size is a crucial aspect of research design. Several factors influence the required sample size, including:
-
Desired level of precision: Higher precision (smaller margin of error) requires a larger sample size.
-
Confidence level: Higher confidence levels (e.g., 99% vs. 95%) require larger sample sizes.
-
Population variability: Higher population variability (larger standard deviation) requires larger sample sizes.
-
Population size: For very large populations, the sample size required is relatively independent of the population size.
Sample size calculations often involve using statistical formulas or software to determine the minimum sample size needed to achieve a desired level of precision and confidence. Power analysis is a common statistical method used to determine the sample size needed to detect a statistically significant effect, if one truly exists.
FAQ Section
-
Q: What is the difference between sampling error and non-sampling error? A: Sampling error arises from using a sample instead of the entire population, while non-sampling error results from flaws in the research design, data collection, or analysis (e.g., measurement error, response bias).
-
Q: How can I reduce non-sampling errors? A: Careful planning, well-trained interviewers, standardized questionnaires, rigorous data validation, and appropriate statistical methods are crucial in reducing non-sampling errors.
-
Q: Is a larger sample always better? A: While larger samples generally reduce sampling error, there's a point of diminishing returns. Increasing sample size beyond a certain point may not significantly improve accuracy while increasing costs and effort.
-
Q: How do confidence intervals help in understanding sampling error? A: Confidence intervals provide a range of values within which the true population parameter is likely to lie, considering the sampling error. A wider confidence interval indicates greater uncertainty due to larger sampling error.
-
Q: What is the role of the standard error in calculating confidence intervals? A: The standard error quantifies the variability of the sample statistic. It is used along with the critical value from the appropriate distribution (e.g., Z or t) to construct the confidence interval.
-
Q: Can sampling error be completely eliminated? A: No, sampling error is inherent in using a sample to estimate population parameters. However, it can be minimized through careful planning and appropriate methodologies.
Practical Tips
-
Clearly define your population: Ensure you have a precise understanding of the target population before selecting a sample.
-
Choose an appropriate sampling method: Select a method that minimizes bias and maximizes representativeness, considering the research question and available resources.
-
Calculate the required sample size: Use statistical methods to determine the appropriate sample size for your research objectives.
-
Use high-quality data collection instruments: Ensure that data collection methods are accurate, reliable, and unbiased.
-
Implement rigorous data quality control: Check for errors and inconsistencies in the collected data, and apply appropriate data cleaning techniques.
-
Report sampling error explicitly: Clearly communicate the margin of error and confidence intervals associated with your findings.
-
Interpret results cautiously: Always consider the possibility of sampling error when interpreting and drawing conclusions from your results.
-
Use statistical software: Statistical software packages can assist in calculating standard errors, confidence intervals, and sample sizes.
Final Conclusion
Sampling errors are an unavoidable aspect of statistical research. Understanding their nature, types, and methods for calculation and minimization is crucial for accurate and reliable statistical inference. By adopting a rigorous approach to sampling design, data collection, and analysis, researchers can mitigate the impact of sampling error and draw more valid and meaningful conclusions from their studies. Further exploration of advanced sampling techniques and error estimation methods will further enhance the precision and reliability of statistical findings across various domains. The continuous pursuit of minimizing sampling error remains a cornerstone of rigorous and dependable statistical practice.
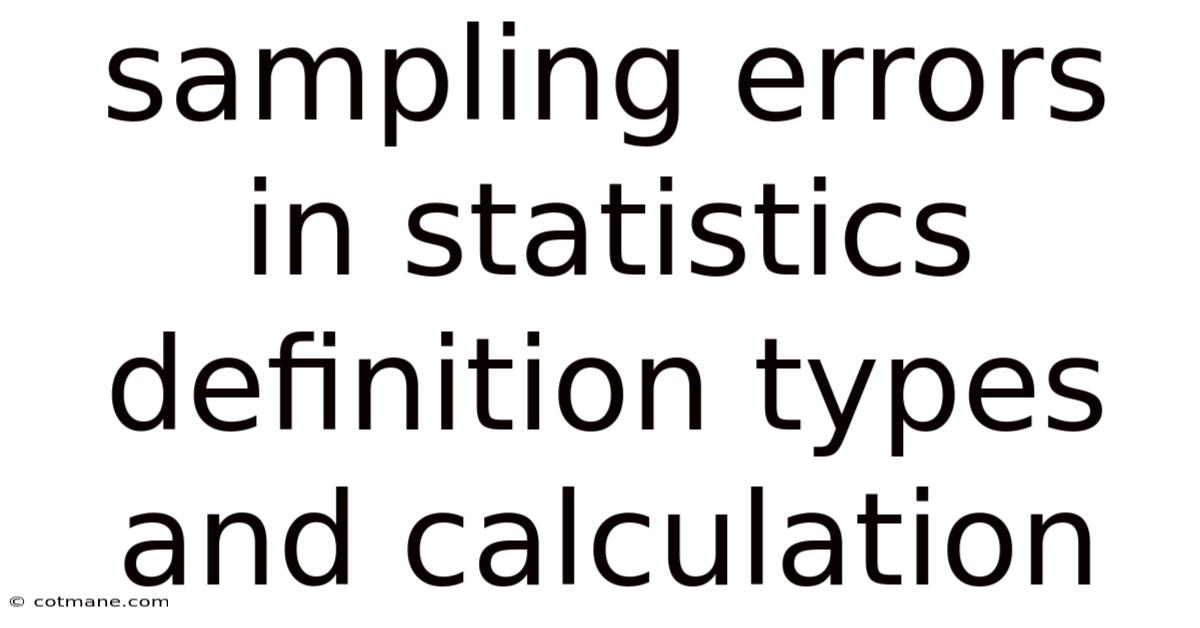
Thank you for visiting our website wich cover about Sampling Errors In Statistics Definition Types And Calculation. We hope the information provided has been useful to you. Feel free to contact us if you have any questions or need further assistance. See you next time and dont miss to bookmark.
Also read the following articles
Article Title | Date |
---|---|
When Someone Dies Who Gets The Retirement Pension Funds | Apr 03, 2025 |
Sp 500 Dividend Aristocrat Index Defined List Of Top Companies | Apr 03, 2025 |
Secondary Mortgage Market Definition Purpose And Example | Apr 03, 2025 |
Self Employment Tax Definition How It Works And How To File | Apr 03, 2025 |
How Do Pension Funds Invest In Infrastructure | Apr 03, 2025 |