Seasonal Adjustment Definition Calculation Methods Example
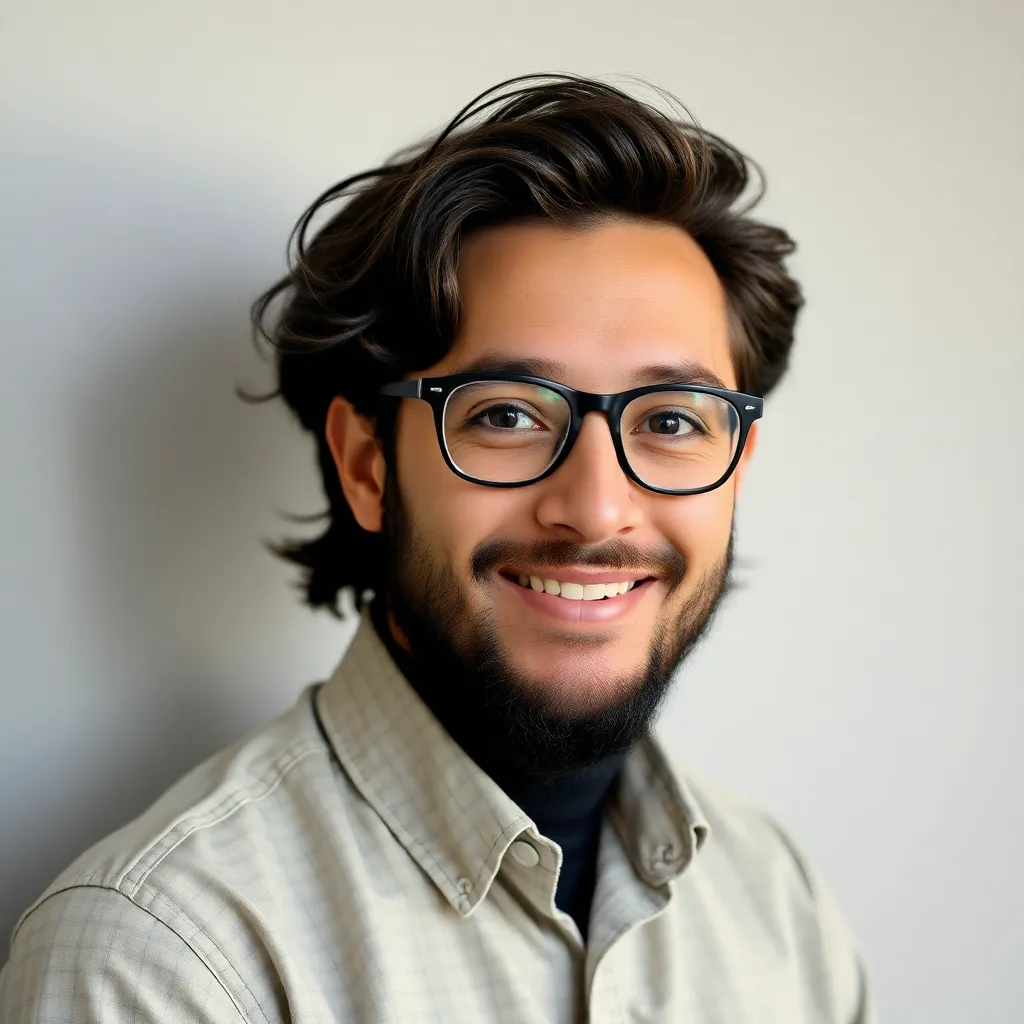
adminse
Apr 03, 2025 · 9 min read

Table of Contents
Unveiling the Seasons: A Deep Dive into Seasonal Adjustment
What makes seasonal adjustment a crucial tool in economic analysis?
Seasonal adjustment is the bedrock of accurate economic forecasting, filtering out cyclical noise to reveal the true underlying trends.
Editor’s Note: This comprehensive guide to seasonal adjustment has been published today, providing up-to-date information and insights into this vital economic analysis technique.
Why Seasonal Adjustment Matters
Economic data, whether it's retail sales, unemployment figures, or industrial production, often fluctuates due to predictable seasonal patterns. For instance, retail sales typically spike during the holiday season, while tourism might peak during summer months. These seasonal variations can obscure the underlying economic trends, making it difficult to assess the true health of the economy. Seasonal adjustment is the process of removing these predictable seasonal fluctuations from raw time series data, revealing a clearer picture of the underlying trend. This allows economists, businesses, and policymakers to make informed decisions based on a more accurate representation of economic performance. Understanding the adjusted data allows for more effective comparison of economic performance across different time periods, eliminating the confounding factor of seasonal variations. Ignoring seasonal effects can lead to inaccurate interpretations of economic changes, potentially resulting in flawed policy decisions and misguided business strategies.
Overview of the Article
This article provides a comprehensive exploration of seasonal adjustment, covering its definition, various calculation methods, and practical examples. Readers will gain a deep understanding of why seasonal adjustment is crucial for accurate economic analysis and how it's applied in real-world scenarios. We will explore both classical decomposition methods and more modern approaches, highlighting the strengths and weaknesses of each. The article culminates in a practical guide to interpreting seasonally adjusted data and offers insights into the potential pitfalls to avoid.
Research and Effort Behind the Insights
This article draws upon extensive research, including seminal works on time series analysis, statistical publications from organizations like the U.S. Census Bureau and Eurostat, and peer-reviewed academic papers on seasonal adjustment techniques. The information presented is based on established statistical methodologies and aims to provide a clear and accurate understanding of the subject matter.
Key Takeaways
Key Concept | Description |
---|---|
Seasonal Adjustment Definition | Removing predictable seasonal variations from time series data to reveal the underlying trend. |
Decomposition Methods | Techniques like classical decomposition, X-11, X-12-ARIMA, and others used to separate seasonal, trend, and irregular components of data. |
Interpretation of Adjusted Data | Understanding that adjusted data reflects the underlying trend, not the raw, unadjusted values. |
Limitations | No method is perfect; some residual seasonality might remain, and extreme events can impact adjustment accuracy. |
Smooth Transition to Core Discussion
Let's delve into the core aspects of seasonal adjustment, starting with a detailed examination of its underlying principles and progressing to an in-depth look at the most commonly used calculation methods.
Exploring the Key Aspects of Seasonal Adjustment
-
Defining Seasonality: Seasonality refers to recurring, predictable fluctuations in a time series that occur within a fixed calendar period, typically a year. This could involve monthly, quarterly, or even weekly variations.
-
Classical Decomposition Methods: These methods, often used as a foundation for more sophisticated techniques, involve separating a time series into its constituent components: trend, seasonal, cyclical, and irregular. The trend represents the long-term direction of the data, the seasonal component represents the regular, periodic fluctuations, the cyclical component represents longer-term fluctuations (typically more than a year), and the irregular component represents unpredictable, random variations. Once these components are identified, the seasonal component is removed to obtain the seasonally adjusted series.
-
X-11 and X-12-ARIMA Methods: These are more advanced statistical techniques developed by the U.S. Census Bureau. X-11 is a widely used method that iteratively estimates the trend, seasonal, and irregular components, refining the estimates in each iteration. X-12-ARIMA builds upon X-11 by incorporating autoregressive integrated moving average (ARIMA) models to better capture the underlying trend and improve the accuracy of the seasonal adjustment.
-
Regression-Based Methods: These methods use regression analysis to model the seasonal component as a function of calendar variables such as month, quarter, or day of the week. The estimated seasonal component is then subtracted from the original time series to obtain the adjusted data.
-
Other Methods and Considerations: Various other methods exist, including those tailored to specific data types or characteristics. The choice of method depends on the nature of the data, the desired level of accuracy, and computational resources. Considerations include outlier detection and treatment, which is crucial as outliers can significantly affect the accuracy of seasonal adjustment.
The Evolution of Seasonal Adjustment Methods
Early methods relied on simple averaging techniques to identify seasonal patterns. The development of X-11 marked a significant advancement, incorporating more sophisticated statistical models to improve the accuracy of seasonal adjustment. The subsequent development of X-12-ARIMA further refined the process by incorporating ARIMA modeling, providing even more accurate and robust results. Recent advancements focus on addressing challenges posed by data with complex seasonal patterns or structural breaks.
Practical Applications of Seasonal Adjustment
Seasonal adjustment is widely used across various fields:
-
Macroeconomic Analysis: Governments and international organizations use seasonally adjusted data to track economic growth, inflation, and unemployment. This allows them to monitor the economy's performance and make informed policy decisions.
-
Business Forecasting: Companies use seasonally adjusted data to forecast sales, production, and inventory levels. This enables them to optimize their operations and make better resource allocation decisions.
-
Financial Markets: Investors and analysts use seasonally adjusted data to assess the performance of companies and markets. This allows them to make more informed investment decisions.
-
Academic Research: Economists and researchers utilize seasonally adjusted data in various studies to analyze economic phenomena and test economic theories.
Future Trends in Seasonal Adjustment
Future developments in seasonal adjustment are likely to focus on:
-
Handling Irregularities: Methods are being developed to more effectively handle unusual events, like pandemics or natural disasters, that can disrupt seasonal patterns.
-
Big Data Integration: Incorporating big data and machine learning techniques may improve the accuracy and efficiency of seasonal adjustment.
-
Real-Time Adjustment: The development of methods that can perform seasonal adjustment in real-time, as data becomes available, will be valuable for timely economic monitoring.
Closing Insights
Seasonal adjustment is an essential tool for accurately analyzing economic data. By removing the influence of predictable seasonal fluctuations, it allows for a clearer understanding of the underlying trends and patterns. The choice of method depends on the specific data and the desired level of accuracy, and understanding the limitations of any method is crucial for proper interpretation. The continuous evolution of seasonal adjustment techniques reflects a commitment to providing even more accurate and reliable economic insights.
Exploring the Connection Between Outlier Detection and Seasonal Adjustment
Outliers—extreme values significantly different from the rest of the data—can significantly distort the estimation of seasonal patterns. These extreme values can artificially inflate or deflate the estimated seasonal component, leading to inaccurate seasonally adjusted data. Robust methods of outlier detection and treatment are therefore crucial. Methods range from simple visual inspection to more sophisticated statistical techniques, like those employed within X-12-ARIMA. The process typically involves identifying potential outliers, assessing their impact on the seasonal adjustment, and either removing or adjusting them before proceeding with the seasonal adjustment. Failure to address outliers can result in misleadingly adjusted data, affecting economic analysis and decision-making. Real-world examples include the impact of a major hurricane on retail sales or a significant policy change affecting unemployment figures. Effective outlier handling ensures that the seasonally adjusted data accurately reflects the underlying economic trends, rather than being skewed by exceptional events.
Further Analysis of Outlier Detection
Outlier Detection Method | Description | Advantages | Disadvantages |
---|---|---|---|
Visual Inspection | Examining time series plots to identify unusual values. | Simple, intuitive | Subjective, prone to errors, especially in large datasets |
Statistical Methods (e.g., Box Plot) | Using statistical measures (e.g., IQR) to identify values beyond a certain range from the median. | Objective, relatively easy to implement | Sensitive to data distribution, may miss subtle outliers |
Time Series Decomposition Methods | Identifying outliers as residuals that are unusually large after removing trend and seasonal components. | Accounts for trend and seasonal patterns | Requires specialized software, computationally more intensive |
Effective outlier detection and treatment enhance the reliability of seasonal adjustment, resulting in more accurate economic analyses and more informed decisions.
FAQ Section
-
What is the difference between seasonally adjusted and unadjusted data? Unadjusted data reflects the raw values, including seasonal variations. Seasonally adjusted data has had these predictable seasonal fluctuations removed, revealing the underlying trend.
-
Why is seasonal adjustment important for economic forecasting? Seasonal adjustment eliminates the confounding influence of seasonal variations, allowing for more accurate predictions of future economic trends.
-
Can seasonal adjustment perfectly remove all seasonal variations? No, some residual seasonality might remain, particularly for data with complex seasonal patterns or infrequent events.
-
What are the potential risks of misinterpreting seasonally adjusted data? Misinterpreting seasonally adjusted data can lead to flawed economic analyses, inaccurate forecasts, and misguided policy decisions.
-
What software packages are commonly used for seasonal adjustment? X-12-ARIMA is widely used, often integrated into statistical software packages like R or specialized time series analysis software.
-
How often should seasonal adjustment be performed? The frequency depends on the data's characteristics but is typically done monthly or quarterly for macroeconomic data.
Practical Tips
-
Understand your data: Before applying seasonal adjustment, thoroughly understand the data's characteristics, including potential outliers and structural changes.
-
Choose the right method: Select a seasonal adjustment method appropriate for your data and the desired level of accuracy.
-
Address outliers: Implement robust methods for outlier detection and treatment before performing seasonal adjustment.
-
Interpret adjusted data cautiously: Remember that adjusted data reflects the underlying trend, not the raw values. Consider both adjusted and unadjusted data for a complete picture.
-
Consult expert advice: If unsure about the appropriate method or interpretation, seek advice from statisticians or economists experienced in time series analysis.
-
Use appropriate software: Employ statistical software packages designed for seasonal adjustment to ensure accurate and efficient processing.
-
Document your methodology: Clearly document the methods and parameters used for seasonal adjustment for transparency and reproducibility.
-
Consider the limitations: Be aware that no seasonal adjustment method is perfect. Some residual seasonality might remain, and extreme events can influence the results.
Final Conclusion
Seasonal adjustment is a critical technique for accurate economic analysis. By carefully removing predictable seasonal fluctuations, it allows economists, businesses, and policymakers to gain a clearer understanding of underlying economic trends. While no method is perfect, the evolution of sophisticated techniques like X-12-ARIMA has significantly improved the accuracy and robustness of seasonal adjustment. Understanding the principles, methods, and limitations of seasonal adjustment is essential for anyone working with economic time series data. Continuous refinement of techniques and increased accessibility to powerful analytical tools promise further advancements in this crucial field, enhancing our ability to accurately interpret and forecast economic activity.
Latest Posts
Related Post
Thank you for visiting our website which covers about Seasonal Adjustment Definition Calculation Methods Example . We hope the information provided has been useful to you. Feel free to contact us if you have any questions or need further assistance. See you next time and don't miss to bookmark.