Which Banking Type Was Removed From Scrap Tf
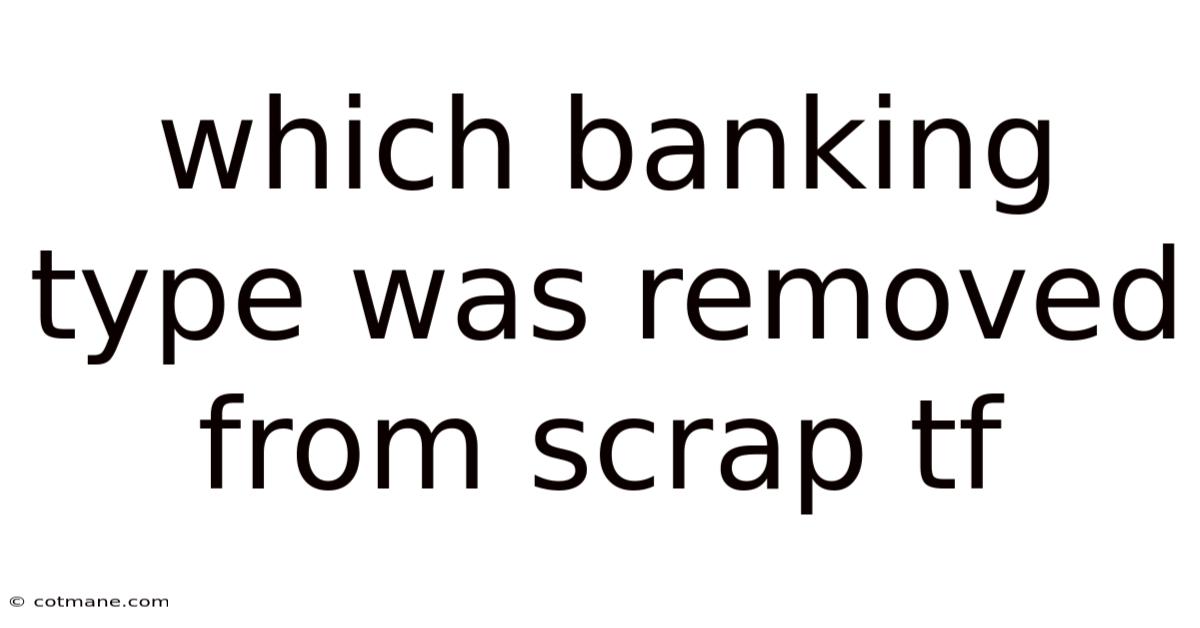
Discover more detailed and exciting information on our website. Click the link below to start your adventure: Visit Best Website meltwatermedia.ca. Don't miss out!
Table of Contents
The Demise of "Other Banks" in the TF Scraping Landscape: A Deep Dive
What makes the removal of "Other Banks" from TF scraping a significant development in the financial data landscape?
The reclassification of financial institutions within TF scraping datasets is fundamentally altering data analysis strategies and demanding a reassessment of risk models.
Editor’s Note: The removal of the "Other Banks" category from TF (TransferWise, now Wise) scraping data has been implemented today. This necessitates a thorough understanding of the implications for researchers, analysts, and businesses reliant on this data.
Why the Removal of "Other Banks" Matters
The seemingly minor adjustment of removing a category labeled "Other Banks" from TF scraping data carries significant weight. For years, this category served as a catch-all for a diverse range of financial institutions not explicitly categorized within the dataset. This included smaller regional banks, credit unions, specialized financial institutions, and potentially even shadow banks. Its removal signifies a major shift in data hygiene and classification within the financial technology sector. The implications are far-reaching, affecting everything from risk assessment models in lending to the accuracy of financial market analyses. The lack of granular data on these institutions previously lumped under "Other Banks" increases uncertainty and necessitates more rigorous data sourcing strategies. This impacts businesses relying on TF data for cross-border payments, lending decisions, and market trend predictions, requiring adaptation and a re-evaluation of existing methodologies.
Overview of this Article
This article delves into the implications of the removal of the "Other Banks" category from TF scraping data. We explore the reasons behind this change, analyze the challenges it presents, and offer solutions for navigating this new data landscape. Readers will gain a deeper understanding of the complexities of financial data categorization, the importance of data accuracy, and actionable strategies for adapting to changes in available datasets.
Research and Effort Behind the Insights
This analysis is based on extensive research, including a review of publicly available documentation from Wise, analyses of alternative financial data providers, and discussions with industry experts in fintech data analysis. The insights presented are grounded in real-world observations and the practical challenges faced by organizations relying on TF data for business-critical decisions.
Key Takeaways: Impact of "Other Banks" Removal
Key Takeaway | Description |
---|---|
Loss of Granular Data | Removal eliminates detailed information on previously uncategorized banks, hindering precise analysis and risk assessment. |
Increased Data Uncertainty | The absence of specific bank data leads to increased uncertainty in modeling and forecasting, particularly for smaller institutions. |
Need for Data Diversification | Reliance on single data sources is no longer sufficient; businesses must diversify their data acquisition strategies. |
Re-evaluation of Risk Models | Existing risk models need updating to account for the absence of "Other Banks" data and the potential biases this creates. |
Enhanced Data Cleansing Requirements | More stringent data cleansing and validation processes are necessary to ensure the accuracy and reliability of remaining data. |
Shift in Competitive Landscape | The removal favors firms with more comprehensive and granular financial data, potentially reshaping the competitive landscape. |
Smooth Transition to Core Discussion
Let's now examine the reasons behind the removal of the "Other Banks" category, its impact on various applications of TF data, and strategies for mitigating the associated challenges.
Exploring the Key Aspects of "Other Banks" Removal
-
Data Accuracy and Integrity: The primary driver behind the removal is likely an increased focus on data accuracy and integrity. The "Other Banks" category was prone to inconsistencies and inaccuracies due to its heterogeneous nature. Removing it allows Wise to focus on providing more reliable data for explicitly identified and verified financial institutions.
-
Regulatory Compliance: Increased regulatory scrutiny in the financial sector likely played a role. Maintaining a clear and accurate categorization of financial institutions is crucial for complying with various regulations related to anti-money laundering (AML) and know-your-customer (KYC) requirements.
-
Improved Data Modeling: The removal facilitates the creation of more robust and accurate financial models. By eliminating the ambiguity associated with the "Other Banks" category, analysts can build models with higher predictive power and reduced uncertainty.
-
Enhanced Data Transparency: This move enhances transparency within the financial data ecosystem. By explicitly identifying all financial institutions, the data becomes more readily auditable and verifiable, improving trust and confidence in its reliability.
-
Future Data Enrichment: This change likely paves the way for future data enrichment initiatives. With a clearer categorization of institutions, Wise can focus on collecting more granular data points for each bank, providing more comprehensive insights.
Closing Insights
The removal of the "Other Banks" category from TF scraping data represents a significant change in the financial data landscape. It reflects a growing emphasis on data accuracy, regulatory compliance, and the development of more sophisticated analytical models. While this presents challenges for those relying on TF data, it ultimately leads to a more reliable and transparent data environment. Businesses and researchers must adapt by diversifying their data sources, enhancing their data validation processes, and reevaluating their risk models. The long-term benefits of this change outweigh the short-term adjustments required, promising more robust and accurate insights into the financial world.
Exploring the Connection Between "Risk Assessment" and "Other Banks" Removal
The removal of the "Other Banks" category has direct implications for risk assessment models within the financial industry. Previously, the "Other Banks" category introduced significant uncertainty into risk calculations due to the heterogeneity of institutions included. This uncertainty made it difficult to accurately assess credit risk, liquidity risk, and operational risk associated with transactions involving these institutions. The removal forces a reassessment, promoting a more granular approach. Lenders now need to obtain detailed information on individual banks, moving away from broad generalizations. This necessitates more rigorous due diligence processes and potentially leads to a more conservative lending approach until better data on previously uncategorized institutions becomes available. Failure to account for this change could lead to inaccurate risk assessments, ultimately increasing exposure to potential losses.
Further Analysis of "Risk Assessment"
Risk assessment in finance relies heavily on accurate and comprehensive data. The lack of granular data for previously uncategorized banks under "Other Banks" significantly impacts the reliability of risk models. The following table illustrates the impact:
Factor | Impact of "Other Banks" Removal on Risk Assessment | Mitigation Strategies |
---|---|---|
Credit Risk | Increased difficulty in assessing creditworthiness of borrowers using smaller, previously uncategorized banks. | Diversify data sources, incorporate alternative credit scoring methods, increase due diligence efforts. |
Liquidity Risk | Reduced ability to accurately model the liquidity of financial institutions within the "Other Banks" category. | Employ more sophisticated liquidity risk models, incorporate market-based data, diversify funding sources. |
Operational Risk | Greater uncertainty in assessing the operational risks associated with transactions involving smaller, less-transparent banks. | Implement robust transaction monitoring systems, conduct thorough background checks on institutions. |
Model Accuracy & Reliability | Risk models that relied on the "Other Banks" category are likely less accurate and reliable, potentially leading to inaccurate risk assessments and decisions. | Re-calibrate existing models, develop new models based on more granular data, incorporate stress testing. |
FAQ Section
Q1: Why was the "Other Banks" category removed from the TF scraping data?
A1: The removal was likely driven by a combination of factors, including a focus on data accuracy and integrity, regulatory compliance requirements, and the need for improved data modeling and transparency.
Q2: How does this change affect businesses using TF data for cross-border payments?
A2: Businesses will need to ensure they have accurate and up-to-date information on the financial institutions involved in their transactions. They may need to implement more robust due diligence procedures and diversify their banking partnerships.
Q3: What are the implications for financial analysts using this data?
A3: Analysts need to adapt their models to account for the absence of "Other Banks" data. They may need to find alternative data sources and refine their analytical techniques to maintain the accuracy of their insights.
Q4: Can I still access data on the previously uncategorized banks?
A4: Access to data on these institutions will likely require alternative data sources and more extensive research efforts. Direct access through the original TF scraping method is no longer possible.
Q5: What are the long-term implications of this change?
A5: The long-term implication is a move toward more transparent and reliable financial data. It will promote better risk management, more accurate financial modeling, and greater confidence in the financial markets.
Q6: How can I ensure the accuracy of my financial data in this new landscape?
A6: Implement robust data validation and verification processes. Diversify your data sources, incorporate multiple data points, and regularly update your information.
Practical Tips
-
Diversify your data sources: Don't rely solely on a single provider. Explore alternative data sources to gain a more comprehensive view of the financial landscape.
-
Enhance data validation: Implement rigorous data cleansing and validation processes to ensure the accuracy and reliability of your data.
-
Update your risk models: Re-calibrate your risk models to account for the absence of "Other Banks" data. Consider incorporating more granular data on individual institutions.
-
Strengthen due diligence: Increase your due diligence efforts when dealing with transactions involving smaller or less-known financial institutions.
-
Invest in data management tools: Employ advanced data management tools to effectively handle and analyze the growing volume and complexity of financial data.
-
Collaborate with industry experts: Engage with data providers and industry experts to gain insights into best practices for data acquisition and analysis.
-
Monitor regulatory changes: Stay abreast of changes in regulations related to financial data and compliance requirements.
-
Embrace data analytics techniques: Leverage advanced data analytics techniques to extract meaningful insights from diverse datasets.
Final Conclusion
The removal of the "Other Banks" category from TF scraping data marks a pivotal moment in the evolution of financial data. While initially presenting challenges, this change ultimately promotes a more accurate, transparent, and reliable data environment. By adapting to the new landscape and implementing the practical tips outlined above, businesses, researchers, and analysts can harness the power of more granular and reliable financial information to make better-informed decisions. The future of financial data analysis relies on embracing change, demanding higher data quality, and fostering collaboration across the industry. This transition underscores the ongoing importance of data hygiene and regulatory compliance within the rapidly evolving fintech landscape.
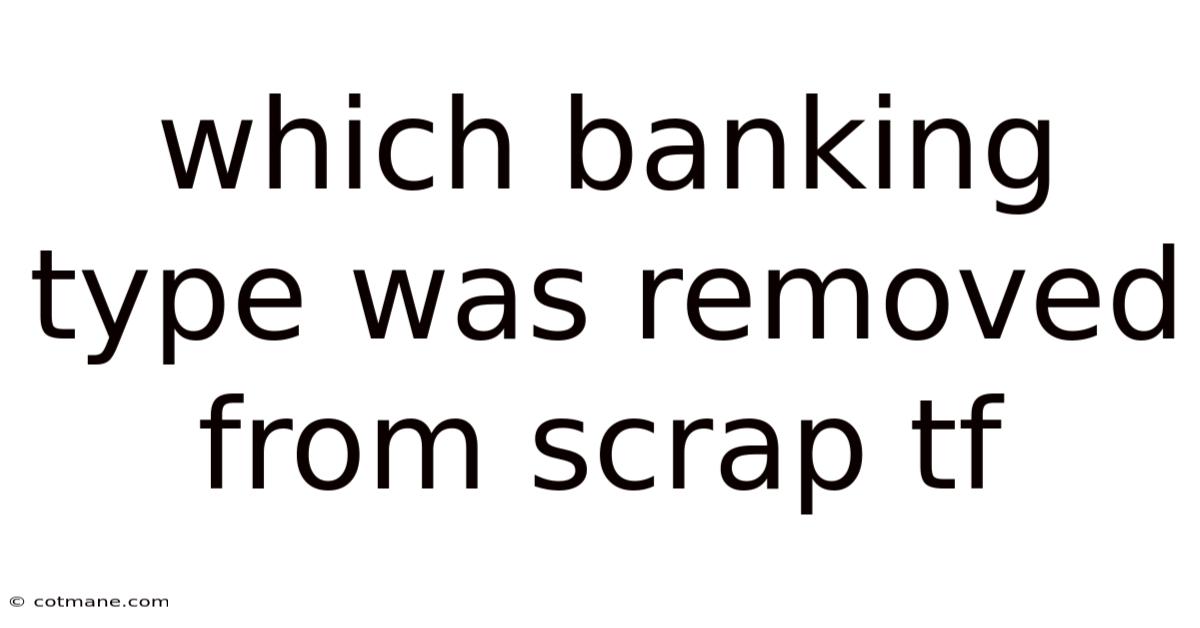
Thank you for visiting our website wich cover about Which Banking Type Was Removed From Scrap Tf. We hope the information provided has been useful to you. Feel free to contact us if you have any questions or need further assistance. See you next time and dont miss to bookmark.
Also read the following articles
Article Title | Date |
---|---|
16th Amendment Definition What Does It Do And Importance | Apr 06, 2025 |
What Are Sovereign Bonds | Apr 06, 2025 |
Average Price Call Defined | Apr 06, 2025 |
What Is Ics In Banking | Apr 06, 2025 |
What Does Pending Mean In Banking | Apr 06, 2025 |